下载中心
优秀审稿专家
优秀论文
相关链接
摘要
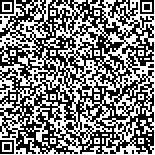
粮食安全、高质量人居环境建设、生物多样性保护、星球健康等社会可持续发展目标和对地球系统的理解、模拟与管理都迫切需要多尺度、长时序、辐射和几何精度高且一致性强的遥感观测数据集和针对用户需求的、信息主题灵活的制图产品。但是由于技术和成本限制,传统的遥感卫星难以提供同时具有高空间分辨率、高时间频率和高质量的观测数据。现有的制图和反演方案多是针对于单一传感器系列,难以充分挖掘和联合利用多源异构遥感大数据的信息潜力,造成观测时段和分辨率有限、时空一致性和可比性较差。因此,遥感领域迫切需要新的技术范式。本文基于前沿的云计算、人工智能、虚拟星座、时空融合重建等技术,针对现有遥感大数据特别是国产卫星数据,提出一套智慧遥感制图(iMap)框架。该框架从用户需求出发、问题驱动,能够大大改善当前遥感数据产品难以满足农林管理、国情监测、生态环境保护、防灾减灾、城市建设等用户的多样化、高精度地表监测需求的现状。在该框架的指导下,基于亚马逊云计算(AWS)高性能、高弹性、可扩展的分布式计算资源,搭建了在线实时、自动化、无服务器、端到端的遥感大数据生产链和并行制图系统,并生产了首套21世纪中国全境逐日无缝数据立方体(SDC)及逐年逐季节土地覆盖和土地利用制图产品。逐日SDC综合利用Landsat和MODIS卫星数据构建虚拟星座,并通过多源时空数据融合重建技术研制得到无云无缝、高精度的反射率产品,作为分析就绪数据(ARD),为高精度定量遥感反演和制图打下根基。基于这一SDC,完成了逐年逐季节地表制图。逐年平均精度超过80%。在制图过程中,基于多套多层土地覆盖和土地利用分类体系,运用有限样本稳定分类理论,迁移使用全季节普适样本库,采用自动机器学习(AutoML)策略集成优化多种分类器,并结合时空一致性变化检测和后处理技术。这两套制图产品证明了本文提出的智慧遥感制图框架的可行性和有效性。未来将进一步完善和发展该框架,以开放和灵活的理念,为促进中国遥感进一步发展提供新的思路。
Sustainable development goals such as food security, high-quality habitat construction, biodiversity conservation, planetary health, and the understanding, modeling, and management of the Earth system urgently require multi-scale, long time series, high-accuracy, and consistent remote sensing observation datasets and mapping products with flexible classification systems to meet user needs. However, due to technical and cost constraints, it is difficult for conventional remote sensing satellites to provide observations with high spatial resolution, high temporal frequency, and high quality at the same time. The existing mapping and inversion schemes are mostly for a single sensor, making it difficult to fully exploit and jointly utilize the information potential of multi-source heterogeneous remote sensing big data, resulting in limited observation periods and resolutions, low spatial and temporal consistency and comparability. Therefore, new technical paradigms are urgently needed in the field of remote sensing. In this paper, based on advanced technologies, including cloud computing, artificial intelligence, virtual constellation, spatio-temporal fusion reconstruction, an intelligent mapping framework is proposed for remote sensing big data. The framework is user-driven and problem-driven, which can significantly improve the current situation that remote sensing data products can hardly meet users’ diversified and high-precision surface monitoring needs in agriculture and forestry management, national monitoring, ecological environment protection, disaster prevention and mitigation, urban planning, etc. Under this framework’s guidance, we built an online real-time, automated, serverless, end-to-end remote sensing big data production chain and parallel mapping system based on Amazon Web Services (AWS) high-performance, elastic, and scalable distributed computing resources. We produced the first set of 21st century daily Seamless Data Cube (SDC) and seasonal to annual land cover and land use mapping products of China. Integrating Landsat and MODIS satellite as a virtual constellation, through multi-source spatio-temporal data fusion and reconstruction technology, the daily SDC, cloud-free, high-precision reflectance product, is developed. As Analysis Ready Data (ARD), it lays the foundation for high-precision quantitative remote sensing inversion and mapping. Based on SDC, we developed the seasonal to annual mapping product with multiple multi-level land cover and land use classification systems, whose mean annual accuracy exceeds 80%. The main mapping pipeline includes migrating the all-season sample set based on stable classification theory with limited samples, optimizing and ensembling multiple classifiers by Automatic Machine Learning (AutoML) strategies, and using change detection and post-processing techniques to achieve consistency. The two sets of products demonstrate the feasibility and effectiveness of the intelligent remote sensing mapping framework proposed in this paper. We will continue to improve and develop the framework with an open and flexible concept to provide new ideas to promote remote sensing development in China.