下载中心
优秀审稿专家
优秀论文
相关链接
摘要
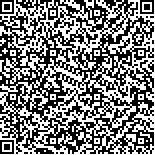
建筑物提取在城市规划等土地利用分析中发挥着重要作用。用于提取建筑物的传统方法通常基于手工特征和分类器,导致精度较低。本文基于编解码结构的卷积神经网络CNN(Convolutional Neural Networks),自主学习多级的和具有区分度的特征来更好地辨识建筑物和背景,实现航空影像中的像素级建筑物提取。该网络由编码子网络和解码子网络两部分组成,编码子网络对输入图像进行空间分辨率压缩,完成特征提取;解码子网络从特征中提升空间分辨率,完成像素级的建筑物提取。此外,本文使用视野增强FoVE(Field-of-View Enhancement)方法减轻边缘现象(切片边缘附近的建筑物提取精度通常低于中心区域附近的精度)的影响,并分别在两个建筑物提取标准数据集上的实验表明,编解码卷积神经网络能有效实现像素级建筑物提取,FoVE能有效提高建筑物提取准确率;通过改变预测时切片大小和重叠度,分析其对建筑物提取结果的影响,揭示了FoVE的饱和性。
Building extraction plays a significant role in land use analysis like urban planning. Classical methods based on hand-crafted features fail to derive prominent building extraction results due to the limited representation capacity of the hand-crafted features. In this paper, we achieve building extraction in pixel level based on a deep Convolutional Neural Network (CNN) with an encoder-decoder structure. In contrast to the hand-crafted features that require professional knowledge and have a poor representation capacity, convolutional neural networks are equipped with a high representation capacity and able to learn highly abstract and distinguishing features from data. The encoder is used to derived a space compressed representation of the input raw image. This compressed representation is also called a feature of the input image and it is assumed to be abstract and distinguishing. The decoder uses the feature as input and recover the space resolution to the size of the input image. Thereby, the encoder-decoder network achieves pixel-wise building extraction in an end-to-end way from the raw image to the building extraction result.Applying the encoder-decoder network to building extraction will cause a Marginal Phenomenon (MP). Specifically, the prediction accuracy near the edges of a patch is usually lower than that near the central area. Marginal phenomenon will lead to the reduction of building extraction accuracy. To alleviate this effect, we propose the usage of the Field of View Enhancement (FoVE) method. The FoVE method includes two parts: enlarging the patch size and cropping patches with overlaps when making predictions. Therefore, the FoVE method contains two hyper-parameters, which are patch size and overlapping size. Extensive experiments on two building extraction datasets are conducted to analyze the impact of the two hyper-parameters through the Precision-Recall Curves (PRC) and some interesting conclusions are derived from the the analysis: (1) Enlarging the input patch size when making prediction can effectively improve the building extraction performance while the improvement saturates as the overlapping size increases; (2) Cropping patches with an overlap when making prediction can improve the building extraction performance while the improvement saturates as the input patch size increases; (3) The FoVE can effectively improve building extraction accuracy but this improvement from the FoVE has a limit; (4) The convolutional neural network for building extraction plays the key role and further attentions should be focused on the network design.In addition to the numerical analysis of the FoVE experimental results, we attempt to explain why FoVE works and why it has a limit. We blame them on the Field of View (FoV) and that is reason why the method is call FoVE. FoV plays an important role in building extraction and a larger FoV is beneficial to building extraction. Firstly, the marginal phenomenon is caused by the lack of context information of the marginal pixels. FoVE improves the overall accuracy through abandoning the unreliable predictions of the marginal pixels. Secondly, enlarging input patches can enlarge the FoV of each pixel and thus improves the accuracy. Thirdly, the the improvement from FoVE has a limit because that when the field of view is large enough, the improvement derived from more contextual information can be ignore.