下载中心
优秀审稿专家
优秀论文
相关链接
摘要
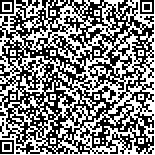
遥感图像数据规模大,光照、遮挡等情况复杂,目标密集、尺度不一以及缺乏大量带标注图像用于训练深度网络等特点对遥感图像分割的完整性和正确性造成了更大的挑战。针对深度卷积网络中因多次卷积造成分辨率显著下降,像素类别预测精度降低的问题,本文在深度卷积编码—解码网络的基础上设计了一个采用完全残差连接和多尺度特征融合的端到端遥感图像分割模型。该模型具有两方面优点:首先,长距离和短距离的完全残差连接既简化了深层网络的训练,又为本层末端融入了原始输入信息,增强了特征融合。其次,不同尺度和方式的特征融合使网络能够提取丰富的上下文信息,应对目标尺度变化,提升分割性能。本文通过对ISPRS Vaihingen和Road Detection数据集做数据扩充并进行实验,分别从平均IOU、平均F1值两方面对模型进行评价。通过与目前先进的模型以及文献中的研究成果进行比较,结果表明本文所提模型优于对比模型,在两个数据集上的平均IOU分别达到了85%和84%,平均F1值分别达到了92%和93%,能够有效提高遥感图像目标分割的完整性和正确性。
Many characteristics of remote sensing images, such as large scale, complex illumination and occlusion, dense, multiple scales, various posture targets, and the lack of a large number of labeled images for training depth networks, pose great challenges to the integrity and accuracy of remote sensing image segmentation. In deep convolutional networks for segmentation, resolution is significantly reduced by multiple pooling, thereby reducing the prediction accuracy of pixel class.On the basis of the deep convolutional coding-decoding network, an end-to-end remote sensing image segmentation model with full residual connection and multiscale feature fusion is proposed in this paper. First, the features in the encoder are merged into the corresponding layers of the decoder, and the residual unit is added to the corresponding convolution layer. The full residual connection constructed by the operation enables the model as a whole to effectively enhance feature fusion and be easier to train. Second, the feature pyramid module, which aggregates multiscale context information, is used on the high-level feature map of the fifth stage of the encoder before feature fusion, thus enabling the model to effectively deal with multiscale changes of the target and improve the segmentation performance.Experiments were conducted on the ISPRS Vaihingen and Road Detection datasets. The proposed model was evaluated from the two aspects of average IOU and average F1-score. A comparison between the current advanced models and the results in the literature shows that the proposed model is better than the comparison models. The average IOU on the two datasets is 85% and 84%, and the average F1 value is 92% and 93%, respectively.An end-to-end remote sensing image segmentation model with full residual connection and multiscale feature fusion is proposed in this paper. The proposed model achieves better results than the current advanced image semantic segmentation model on the two datasets. The segmentation targets are more complete, continuous, and have fewer misclassifications and leakages. The proposed model also achieves better results than the comparative model in road segmentation of remote sensing images from different sources, thereby further verifying the robustness of the model.