下载中心
优秀审稿专家
优秀论文
相关链接
摘要
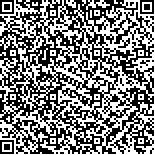
遥感影像建筑物提取具有重要的应用价值。然而,高分辨率遥感影像中细节信息繁多、特征复杂,增加了建筑物提取难度。针对这一问题,本文提出一种基于多尺度SLIC-GMRF和FCNSVM的建筑物提取方法,一定程度上提高了高分辨率遥感影像建筑物提取能力。首先,利用多尺度SLIC-GMRF分割算法确定初始建筑物区域,然后,充分利用FCN神经网络在语义分割中的优势抽取建筑物特征,最后,结合提取出的建筑物特征训练SVM分类器细化建筑物提取结果,通过3种控制实验,两种对比方法得出以下结论:SLIC分割算法影响初始分割结果;SVM分类器影响建筑物细部提取;FCN特征影响SVM分类器性能。对于特征清晰、遮挡干扰较少的研究区,本文方法能够较好提取影像中的建筑物,查准率、查全率、质量指标均优于对比方法,对建筑物复杂分布的研究区同样能够取得较好的提取效果。
The extraction of buildings from remote sensing imagery has an important application value. However, high-resolution images contain detailed information and complex features that hinder the difficulty of building extraction process. To address this problem, we propose a building extraction method of building extraction based on multi-scale SLIC-GMRF and FCNSVM that demonstrates an improved ability of extracting buildings from high-resolution remote sensing images to some extent. First, a multi-scale SLIC-GMRF segmentation algorithm is applied to determine the initial building area, and then the advantages of the FCN neural network in semantic segmentation are utilized to extract the building features. Second, the extracted building features are used to train an SVM classifier to refine the building extraction results of building. The results of three control experiments and two comparative tests reveal that the SLIC segmentation algorithm affects the initial segmentation results, the SVM classifier affects the extraction of building details, and the FCN features influence the performance of the SVM classifier. The precision rate, recall rate, and quality index of the proposed method are all better than the compared methods. The following conclusions can be drawn from the experimental results. For the study area with clear features and minimal obstructions, the proposed method can effectively extract buildings from an image. This method can also obtain ideal results for areas with a complex distribution of buildings can also get ideal results.