下载中心
优秀审稿专家
优秀论文
相关链接
摘要
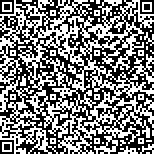
为利用高分辨率遥感影像实现高精度的飞机目标变化检测,提出了一种自适应的多特征融合变化检测与深度学习相结合的方法。首先,通过加权迭代的多元变化检测法获取变化强度图,并结合自适应的直方图统计法自动获取显著的变化与不变化样本;然后,提取多时相影像的光谱、边缘和纹理特征,完成多特征融合的变化检测,并通过形态学处理得到变化图斑;最后,利用训练的NIN(Network in Network)结构的卷积神经网络飞机识别模型,完成变化图斑的类型判别,实现变化飞机的检测。实验结果表明,本文方法在两组数据的正确率分别达到100%和91.89%,均优于对比方法,能实现准确可靠的飞机目标变化检测。
Multi-feature fusion has been widely employed for high-resolution remote sensing images change detection given its ability to reduce the influence of radiation difference, projection errors, and shadows. However, most multi-feature fusion methods depend on artificially designed fusion rules or man-made samples. Meanwhile, many methods for target change detection on bi-temporal images have successfully detected the changed areas yet fail to recognize the number and location of changed targets. To address these limitations, this paper proposes an aircraft target change detection method for high-resolution remote sensing images that combines adaptive multi-feature fusion for change detection with deep learning for target recognition. First, Iteratively Reweighted Multivariate Alteration Detection (IR-MAD) is applied to generate a change intensity map, and then adaptive histogram statistics are employed to calculate the threshold for the automatic acquisition of conspicuous changed and unchanged samples. Second, the edge and textural features are extracted respectively from multi-temporal images, and a multi-feature fusion change detection is carried out by using a support vector machine and the previous samples. The change polygons are obtained after morphological processing. Third, to prevent a false detection by a single threshold, a multi-threshold strategy is adopted, and raw images from the change polygons are recognized by using the aircraft recognition model that is trained by a few plane slices and the convolutional neural network with network in network. The experiments for airport images change detection and final aircraft target change detection experiments are performed using two datasets. First, we compare our proposed method is compared with other thresholds and check whether to use multi-features should be used to highlight the effectiveness of our multi-feature fusion change detection method with adaptive samples. To verify its performance, we compare our method with some popular change detection methods are compared, including Multivariate Alteration Detection (MAD), IR-MAD, principal component analysis, change vector analysis, robust change vector analysis, and iterative slow feature analysis. The experiments show that the overall accuracy of our proposed change detection method outperforms the other compared methods in terms of accuracy and false alarm rate. We obtain our target change detection results based on the change map, and validate the excellent performance of our proposed method based on its accuracy. To fully use of the spectral, spatial, and textural features of high-resolution remote sensing images, we design an adaptive multi-feature fusion method for change detection that requires less manual work and reduces the influence of radiation difference, projection error, and shadows. We also propose an aircraft target change detection method by combining the multi-feature fusion change detection with target recognition using deep learning. The experimental results validate the excellent performance and reliability of our method.