下载中心
优秀审稿专家
优秀论文
相关链接
摘要
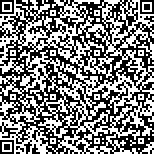
在无需先验标签样本的情况下,非监督降维可以有效简化高光谱图像的特征空间,避免目标分类中的霍夫效应。本文提出JM非线性变换优化的自适应降维模型来研究面向图像目标分类的高光谱波段选择问题。该方法考虑波段的信息量和独立性等两个重要因子,针对其测度方法的差异性问题,引入JM变换函数进行规范化优化。选用线阵高光谱和面阵显微光谱等两个图像数据集,在k最邻近和随机森林分类器下,进行了多组监督分类实验,结果表明,在Kappa系数、总体分类精度和平均分类精度上,本文方法均优于3种非监督方法MABS、InfFS和LSFS。说明本文提出的JM变换的自适应降维模型能够有效降低特征维度,满足高光谱图像分类的高精度要求。
Hyperspectral remote sensing images, which collect rich spectral and spatial information of observed targets, usually contain dozens to hundreds of narrow bands with wavelengths ranging from the visible light region to the near-infrared spectra. With such an abundant number of spectral features, hyperspectral images (HSI) allow us to distinguish different of objects or targets by rule and line. Unfortunately, such high-dimensionality data pose a challenge in data transmission, storage, and processing. Specifically, those HSIs with high redundancy information and strong correlation are prone to a Hughes phenomenon during the image classification process. Therefore, dimensionality reduction is necessary for targets classification. Moreover, without using prior label samples, unsupervised dimensionality reduction can effectively simplify the HSI feature space, and prevent the Hughes phenomenon in the targets classification. In this paper, the Jeffries–Matusita (JM) modified adaptive band selection (JM2ABS) method is proposed to extract proper features from HSI datasets. Generally speaking, a band that contains many information and demonstrates strong independence is a very important feature that helps unsupervised band selection methods to classify targets. The JM2ABS method considers both the information capacity and independence of HSI bands. Given the significant differences in the measurements of a band’s information capacity and its independence, we introduce the JM transform function to normalize the distributions of the information capacity and the independence of HSI data. Thus JM2ABS shows that both the information capacity and the independence are equally important in unsupervised dimensionality reduction. We also compare our proposed JM2ABS method against three typical methods, namely, the modified adaptive band selection method, the Laplacian score feature selection method, and the infinite feature selection method. By using random training samples, we perform supervised classification experiments on two kinds of HSI public datasets (linear and planar arrays). The results demonstrate that JM2ABS outperforms the other three typical methods in terms of Kappa value, overall classification accuracy, and average classification accuracy. Moreover, under a small number of bands, JM2ABS can reach a high and stable level regardless of the different datasets and different classifiers used. The proposed JM2ABS can select the proper features of HSI datasets for their classification application. The JM transform function is a kind of nonlinear distribution function that can standardize variables from different scales to the same. To demonstrate the feasibility of JM transform optimization, we set the same weight for the information content and the independence. In our future work, we will explore the similarities and differences between the information capacity and the independence in dimensionality reduction.