下载中心
优秀审稿专家
优秀论文
相关链接
摘要
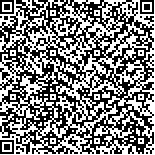
高光谱压缩感知(HCS)对于解决机载或星载高光谱数据的存储与实时传输具有重要意义。目前,线性混合模型(LMM)已被成功应用于HCS;然而,由于光照条件、地形变化以及大气作用等的影响,所获取的地物光谱会发生扰动,从而限制了HCS重建质量的提高。在LMM基础上,通过引入光谱修正项来修正光谱扰动,提出了光谱扰动修正的LMM(SPC_LMM);在此基础上,进一步提出了基于SPC_LMM的HCS(HCS_SPC_LMM)方法。该方法在采样端仅对原始高光谱图像进行光谱维压缩采样,基于压缩采样数据,将SPC_LMM应用HCS的重建,利用交替方向乘子法(ADMM)分别估计SPC_LMM中各分量的最优值,以获得最优的高光谱图像重建质量。实验结果表明,HCS_SPC_LMM能够获得优于其他典型HCS方法的重建质量。
Hyperspectral Compressed Sensing (HCS) is crucial for data storage and the real-time transmission of airborne- or spaceborne-based imaging platforms. The Linear Mixing Model (LMM) has been successfully applied to HCS reconstruction. However, the obtained spectrum may be disturbed, thereby limiting the improvement of reconstruction quality due to the influence of illumination conditions, topographic changes, and atmospheric effects. Spectral disturbance is corrected on the basis of LMM by introducing the spectral correction term, and a linear mixing model for spectral perturbation correction is proposed. Moreover, an improved HCS method based on modified LMM is proposed. This proposed model only performs spectral compressed sampling on the original hyperspectral images at the sampling end. The proposed method uses the proposed spectral perturbation correction model to reconstruct the original hyperspectral images based on the compressed sampling data. The alternating direction multiplier method is used to estimate the optimal values of each component in the modified LMM to obtain the optimal reconstruction quality. Experimental results show that the proposed method can achieve better reconstructed performance compared with other classical HCS methods.