下载中心
优秀审稿专家
优秀论文
相关链接
摘要
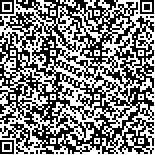
多尺度分析技术广泛应用于高分辨率遥感影像的特征提取和建模。分解层数受制于影像的大小,下采样小波变换实现的影像多尺度表达难以描述大范围的空间模式,导致分类结果出现“胡椒盐”现象;面向对象的影像分析技术虽避免了“胡椒盐”现象,但由于仅利用了单尺度的的特征,也难以描述影像多层次的空间模式,导致分类精度较低。为改善分类结果中的“胡椒盐”现象和提高分类精度,提出了一种结合区域多尺度遥感影像分割和马尔可夫随机场的分类方法。首先,获得原始影像过分割区域,依据区域内亮度均值以及区域间的共享边界长度信息,提取影像低频和高频特征,采用该低频特征波段代替原始影像,重复分割与特征波段提取过程,形成影像的区域多尺度表达。然后,以原始图像为初始尺度,以分割区域为处理单元,以更细尺度分类结果为标记场先验,以当前高频特征建立特征场,逐层分类、投影,获得最终尺度分类结果。合成纹理影像和多光谱遥感影像的实验表明:相比于小波域多尺度建模方法和单尺度区域建模方法,本文提出的方法可以有效提高分类精度,并避免“胡椒盐”现象的产生。
Multiscale analysis technique can describe an image from different resolutions and has been widely used for extracting features and modelling of remotely sensed images. The subsampled wavelet transforms are commonly employed for establishing the multiscale representation of an image. However, the wavelet-based features cannot describe patterns with long spatial spans and often result in noisy classification results. By contrast, the object-based image analysis can create classification maps composed of compact land objects. However, features extracted from a single scale still cannot provide discriminating information for the land cover classification. To improve the classification accuracy and alleviate noisy thematic maps, a regional multiscale classification method is proposed.The proposed method consists of two main blocks, including establishing a regional multiscale image representation and classifying on the basis of Markov Random Field (MRF). In the first block, the mean shift segmentation method is employed to create initial over-segmented regions. Thereafter, a rule combining the grey values in the regions and the shared boundary lengths among regions is designed to extract the low-frequency part of an image. The current high-frequency part is obtained by subtracting the original image and the current low-frequency portion. A regional multiscale representation can be iteratively established by replacing the original image with the low-frequency part and repeating the segmenting and decomposing process. In the second block, the classification result obtained from the original image is considered the prior of the label field in the first decomposed level, and the high-frequency part in the first level models the feature field of MRF. The classification result in the first level is obtained by solving an objective function consisting of feature and label energies. By iteratively projecting the current classification result to the next level and modelling the feature field with the high-frequency part, the final classification map can be acquired in the coarsest scale.To examine the effectiveness of the proposed method, three groups (six images in total), such as Prague textures, synthetic remote sensing textures, and QuickBird multispectral images, are adopted. The proposed regional multiscale MRF (RMRF) is compared with the Iterated Conditional Model (ICM), Graph Cut (GC), and Wavelet-based MRF classification methods (WMRF). Visual inspection and quantitative measurement are employed for the comparison. As GC fails to integrate the spatial information among neighbouring pixels, the classification results are dominated by the pixel spectral values. Textures or land cover patterns with complex spectral heterogeneity cannot be properly captured. WMRF provides a pixel-level multiscale representation, which is also insufficient for describing texture patterns across a large spatial span. ICM, GC and WMRF may create noisy thematic maps with different extensions when the spectral heterogeneity of a given land cover pattern is extreme. On the contrary, RMRF has a flexible framework to extract and model spatial information. RMF can capture the essential features of land objects with large spectral heterogeneity, resulting in maps with less noise.This study proposes an RMRF for classification. Experiments demonstrate that the established multiscale representation can efficiently describe texture patterns with wide spatial spans, such as land objects with complex structures. By combining the multiscale representation with MRF model, RMRF can achieve a high-precision semantic segmentation of ground objects. The adaptive estimation of the decomposition layer number and parameters of the algorithm are the on-going works.