下载中心
优秀审稿专家
优秀论文
相关链接
摘要
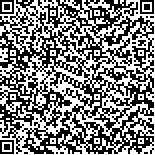
针对高分四号卫星图像中波红外谱段空间分辨率远低于相应的可见光近红外谱段,提出一种基于深度学习的高分四号卫星图像中波红外谱段超分辨率重建方法。首先,设计卷积回归网络进行初步重建,利用8倍下采样的可见光近红外谱段和原始中波红外谱段训练回归网络。然后,设计卷积重建网络进行进一步重建,利用低分辨率初步重建结果和原始中波红外谱段训练重建网络。最后,将原始可见光近红外谱段依次输入到训练好的回归网络和重建网络,得到最终的中波红外谱段超分辨率重建结果。以湖北和江西部分地区真实数据进行试验,结果表明该方法能有效提高中波红外谱段空间分辨率,与其他方法对比均方根误差至少下降了7.54,且目视效果更清晰自然,有利于扩展高分四号卫星的应用范围。
GF-4 is a geostationary orbit satellite launched under the support of the major special project of China’s high-resolution earth observation system. Notably, the spatial resolution of the medium-wave infrared channel in GF-4 satellite imagery is much lower than the corresponding visible near-infrared channels of the same scene. The resolution of the medium-wave infrared channel needs to be improved.In this study, a deep learning method termed as GF-4 Super-Resolution Network (GF-4-SRN) is proposed for super-resolution GF-4 satellite imagery. First, a convolutional regression network is designed for preliminary reconstruction of the original image. The convolutional regression network is trained using eight times down-sampled visible near-infrared channels and the original low-resolution medium-wave infrared channel. Thus, the correspondence between the visible near-infrared channels and the medium-wave infrared channel is developed. Second, a convolutional reconstruction network is designed to further reconstruct the preliminary results. This network is trained using the low-resolution preliminary reconstruction results and the original medium-wave infrared image. Notably, the convolutional reconstruction network can build the relationship between the preliminary reconstruction results and the medium-wave infrared image. Finally, the original visible near-infrared channels in GaoFen-4 satellite imagery are fed into the trained GF-4-SRN. The final super-resolution reconstruction results can be obtained by the relationship between visible near-infrared images and the medium-wave infrared image.Experiments are performed on two real-world GF-4 satellite images acquired from Hubei and Jiangxi regions. Experimental results demonstrate that the proposed GF-4-SRN method can effectively enhance the spatial resolution of the medium-wave infrared image. Compared with the root mean square error of state-of-the-art methods, that of the GF-4-SRN is reduced by at least 7.54. Moreover, the visual effects are much clearer and more natural. Therefore, the GF-4-SRN contributes to expanding the application range of the GF-4 satellite imagery.This paper proposes a deep learning-based super-resolution method for GF-4 satellite imagery. The proposed GF-4-SRN is composed of preliminary reconstruction by convolutional regression network and further reconstruction by convolutional reconstruction network. Experimental results on Hubei and Jiangxi regions demonstrate the effectiveness of the proposed GF-4-SRN. The spatial resolution is increased by fully utilizing the information provided by the visible near-infrared images. The root mean square error, erreur relative globale adimensionnelle de synthèse, and spectral angle mapper are lower than those of other methods. Meanwhile, the peak signal-to-noise ratio is higher than those of the competitors. Moreover, the visual effect of the results provided by the GF-4-SRN is much clearer and the reconstruction results contain more details. Notably, the deep learning-based method has great potential in super-resolution GF-4 satellite imagery.In the future, we will improve the GF-4-SRN by adopting various window sizes in the convolutional kernel. The network connection mode and optimization method can also be changed to improve the speed and performance of the network. The GF4-SRN will also be extended to other application areas, such as disaster monitoring.