下载中心
优秀审稿专家
优秀论文
相关链接
摘要
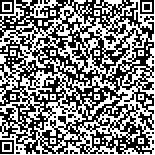
利用极化合成孔径雷达(PolSAR)能够实现地物的识别和分类,而多时相全极化SAR可以获取地物更多的散射特征,提升地物识别精度,但高维散射特征的引入会带来严重的维数灾难问题。为了实现对高维散射特征的有效降维,本文提出一种基于栈式稀疏自编码网络S-SAE(Stacked Sparse AutoEncoder)的多时相PolSAR散射特征降维方法。该方法首先对PolSAR数据进行极化目标分解以获取高维散射特征;然后使用S-SAE对获取的多维特征进行降维处理,其中S-SAE降维方法首先采用无监督训练方式进行逐层贪婪训练;再结合Sigmod分类器,利用监督训练的方式对S-SAE进行参数优化,实现高维特征的有效降维;最后以降维后的特征作为支持向量机(SVM)和卷积神经网络(CNN)分类器的输入,实现地物分类。通过仿真和实测的两组多时相Sentinel-1数据处理结果表明,双隐层的S-SAE降维方法在各分类器上均取得最优的降维效果;对比各降维方法在SVM分类器上的分类精度,S-SAE较于局部线性嵌入(LLE)与主成分分析(PCA)降维方法,总体分类精度分别至少提升了9%和14%;在CNN分类器上,S-SAE较于LLE与PCA降维方法,总体分类精度分别至少提升了7%和9%。
Polarimetric Synthetic Aperture Radar (PolSAR) has been proven to recognize and classify various ground objects, and multitemporal fully PolSAR can acquire many scattering features to improve the accuracy of recognition and classification. However, the decomposed scattering features with high dimensionality can cause serious problems of “curse of dimensionality.” This paper proposes a multitemporal PolSAR scattering feature dimension reduction method based on Stacked Sparse AutoEncoder (S-SAE) to effectively reduce the dimensionality of high-dimensional scattering features. The proposed method firstly decomposes the PolarSAR data to obtain high-dimensional scattering features and adopts S-SAE to reduce the dimensionality of the acquired multidimensional features. For the S-SAE construction, unsupervised layer-by-layer greedy training is performed to optimize the main parameters. Combined with a sigmoid classifier, the parameters of S-SAE are finely tuned through supervised training to achieve effective dimension reduction of high-dimensional features. The reduced low-dimensional features are taken as the input of Support Vector Machine (SVM) and Convolutional Neural Network (CNN) classifiers to evaluate the performance of feature dimension reduction. The performance of the proposed method is validated on two datasets of multitemporal simulated and real Sentinel-1 data. Experimental results show that the S-SAE method with two hidden layers achieves the best performance of feature dimension reduction. Compared with traditional Locally Linear Embedding (LLE) and Principal Component Analysis (PCA) dimension reduction methods, the overall classification accuracy of S-SAE for the SVM classifier is raised by at least 9% and 14%. The overall classification accuracy of S-SAE for the CNN classifier is at least 7% and 9% higher than that of LLE and PCA, respectively.