下载中心
优秀审稿专家
优秀论文
相关链接
摘要
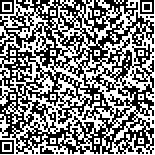
利用小型无人机进行遥感图像配准在自然灾害损害评估、环境监测和目标检测与追踪等领域发挥着至关重要的作用,但小型无人机的图像采集过程容易受风速/风向、复杂地形、电池容量、飞行姿态、飞行高度等自然或人为因素的影响。这些问题通常会导致捕捉到的场景重叠率低与图像非刚性畸变,在特征点提取过程中产生大量冗余点,增加了图像配准的难度。本文提出一种基于特征点的小型无人机图像配准方法,该方法的核心思想是在配准过程中识别冗余点,同时最大化可用内点数量。所识别的冗余点当作控制点,用于控制网格代图像的运动。最后通过最大化内点和合理移动控制点来恢复图像变换。本文使用50对小型无人机图像进行特征匹配和图像配准的实验,其中平均配准精度可达80.38%,并且本文方法在所有的情况下都优于5种当前流行算法。
Remote sensing image registration using small unmanned aerial vehicles plays a significant role in military and civilian fields, such as natural disaster damage assessment, ground change detection, environmental monitoring, military damage assessment, and ground target identification. This technique aims to align two or more images (i.e., the sensed image and the reference image) of the same scene captured from different viewpoints, from different times or with different sensors. However, the imaging perspective of small unmanned aerial vehicles is vulnerable to wind speed/direction, complex terrain, battery capacity, aircraft posture, flying height, and other human factors. Such issues often cause nonrigid distortions and low overlap ratios within the captured scenes, thereby generating severe outliers during feature point extraction. Two major types of classification, namely, (1) area-based methods and (2) feature-based methods, are found in accordance with the methodological differences in current methods. We mainly focus on developing a feature-based method in this work. We introduce and discuss the current methods during the classification.In this work, the key idea of the proposed method is to maintain a high matching ratio on inliers while using outliers for image registration. The main contributions of the proposed method are: (1) a relatively low initial threshold, which is lower than the default Scale-Invariant Feature Transform (SIFT) threshold, is usually used to extract two large sets of feature points. A putative control point processing strategy is then designed to gradually identify outliers and maximize the number of reliable inlier pairs. Dynamic SIFT threshold helps to build a coarse-to-fine transformation; (2) a local spatial structure similarity preservation is proposed to constrain the local structure of putative inliers during registration while using a global constraint to refine the warping field by coherently moving putative dummy control points. (3) a dynamic Gaussian kernel is developed to control the displacement distances of feature points such that the transformation is gradually changed from rigid to nonrigid for assisting the above coarse-to-fine search strategy.This study considers five groups of multiview small unmanned aerial vehicle images of typical landform in different regions as the study area. The experiments on feature matching and image registration are performed using 50 pairs of small unmanned aerial vehicle images. Compared with five state-of-the-art methods (SIFT, SURF, CPD, GLMATPS, and GL-CATE), our method demonstrates higher registration quality in all scenarios, where the viewpoint change is up to 100°, and the overlap rate is close to 0.5.In this work, we presented a feature-based method for the remote sensing image registration of small unmanned aerial vehicles based on inlier maximization and outlier control. The key idea is to gradually identify the control points and maximize the number of available inlier pairs. The identified control points are simultaneously used to refine the warping grids within the overlap and nonoverlap areas by reasonably and coherently moving them. The image transformation is recovered by the maximized inliers and the reasonably moved control points. Extensive experiments proved that the use of outliers can improve image registration accuracy.