下载中心
优秀审稿专家
优秀论文
相关链接
摘要
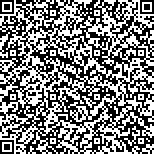
随着卫星遥感技术的发展,越来越多的卫星观测数据被应用于预测海洋内部温盐结构信息,而如何有效提高海洋内部温盐信息的预测精度仍是一个挑战。本文应用LightGBM算法结合随机森林算法构建全球海洋次表层(0—1000 m)温度异常(STA)与盐度异常(SSA)的预测模型,模型使用海表卫星观测数据(海表高度异常(SSHA)、海表温度异常(SSTA)、海表盐度异常(SSSA)和海表风场异常水平和垂直分量(USSWA、VSSWA),结合经纬度信息(LON、LAT)作为预测变量,使用Argo次表层温盐数据作为模型训练与测试标记。本文使用五参数模型(SSTA、SSHA、SSSA、USSWA、VSSWA)、带纬度六参数模型(LAT、SSTA、SSHA、SSSA、USSWA、VSSWA)、带经度六参数模型(LON、SSTA、SSHA、SSSA、USSWA、VSSWA)与带经纬度七参数模型(LON、LAT、SSTA、SSHA、SSSA、USSWA、VSSWA)来着重分析LON与LAT在STA、SSA遥感预测中发挥的作用。结果表明LON与LAT在STA、SSA各自预测中发挥不同的作用。在单时相和时序预测STA中LON与LAT对模型的贡献随着深度的增加逐渐增大,而在单时相和时序预测SSA中LON与LAT对不同深度预测模型始终保持较大的贡献。在单时相预测STA与SSA中LON较LAT对模型贡献更大,而在时序预测STA与SSA中LAT较LON对模型贡献更大。经纬度信息是全球海洋次表层温盐机器学习预测的重要参数,可以提高模型的预测精度。同时,LightGBM较随机森林在预测海洋次表层温盐异常时精度更高鲁棒性更强。
Satellite observation data are increasingly used to derive and predict thermohaline information within the ocean due to the development of satellite technology. However, the effective improvement of prediction accuracy in satellite remote sensing is still challenging. A model with strong spatiotemporal applicability and high robustness for subsurface thermohaline estimation is necessary due to the complex and highly dynamic processes in the ocean.In this study, a new LightGBM method combined with random forest algorithm is used to predict global subsurface temperature and salinity anomalies in the upper 1000 m depth based on remote sensing and Argo float data. The proposed method uses multisource sea surface parameters, including Sea Surface Height Anomaly (SSHA), Sea Ssurface Temperature Anomaly (SSTA), Sea Surface Salinity Anomaly (SSSA), and northward and eastward components of sea surface wind anomaly (USSWA and VSSWA), combined with longitude and latitude data (LON and LAT) as predictor variables and Argo gridded data as training and testing labels for model construction and prediction. This study creates five-parameter model (SSTA, SSHA, SSSA, USSWA, and VSSWA), six-parameter model with latitude (LAT, SSTA, SSHA, SSSA, USSWA, and VSSWA), six-parameter model with longitude (LON, SSTA, SSHA, SSSA, USSWA, and VSSWA), and seven-parameter model with longitude and latitude (LON, LAT, SSTA, SSHA, SSSA, USSWA, and VSSWA) to analyze and evaluate the role of LON + LAT in STA and SSA prediction using LightGBM and RF models.Using the monotemporal LightGBM model to predict STA, the average R2 of the seven-parameter model, six-parameter model with latitude, six-parameter model with longitude, five-parameter model is 0.980, 0.922, 0.937, 0.776 and the average RMSE is 0.072 ℃, 0.141 ℃, 0.127 ℃, 0.240 ℃. The average R2 in the SSA prediction is 0.963, 0.846, 0.872, 0.545, and the average RMSE is 0.012 psu, 0.025 psu, 0.022 psu, 0.042 psu. The average R2 of the seven-parameter model, six-parameter model with latitude, six-parameter model with longitude, five-parameter model when using time-series LightGBM model to predict STA is 0.703, 0.655, 0.585, 0.523, and the average RMSE is 0.298 ℃, 0.317 ℃, 0.356 ℃, 0.378 ℃. The average R2 in the SSA prediction is 0.426, 0.277, 0.197, 0.103, and the average RMSE is 0.050 psu, 0.057 psu, 0.059 psu, 0.064 psu. Hence, the seven-parameter model demonstrated the best performance. The maximum R2 and minimum RMSE in the seven-parameter LightGBM model are 0.992, 0.981 and 0.022 ℃, 0.004 psu in the monotemporal STA, SSA prediction. Meanwhile, the maximum R2 and minimum RMSE are 0.817, 0.574 and 0.092 ℃, 0.013 psu in the time-series STA, SSA prediction. The prediction accuracy of the model decreases gradually with increasing depth.This study suggested that LON + LAT significantly contribute to both STA and SSA prediction, but differently impact on respective STA and SSA prediction. The contribution of LON + LAT to the model increases with depth in the monotemporal and time-series STA prediction while maintaining a large contribution to the model at different depths in the monotemporal and time-series SSA prediction. LON makes a larger contribution than LAT in the monotemporal STA and SSA prediction, while LAT plays a more significant role than LON in the time-series STA and SSA prediction. Furthermore, LightGBM outperforms RF and is more robust in the subsurface thermohaline prediction.