下载中心
优秀审稿专家
优秀论文
相关链接
摘要
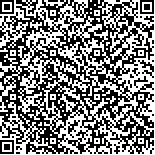
红边作为植被敏感波段,其红边特征的运用是遥感识别农作物并实现精准农业的高新手段之一。以黑龙江松嫩平原北部为研究区,以国内首个提供红边波段的多光谱高分六号影像和玉米、大豆、水稻总计82859个作物样本同时作为研究对象,从以下几个方面研究了红边波段和红边指数波段等红边特征在农作物识别中的表现,并评估了农作物的识别精度。(1) 通过作物样本辐射亮度值的统计特征,初步显示了在两红边波段0.710 μm和0.750 μm处有比其他波段更好的区分;(2) 根据传统归一化植被指数形式构建了红边归一化植被指数NDVI710和NDVI750,综合两指数在J-M距离表征的作物样本类别区分度上比传统NDVI更显著;(3) 通过多种手段筛选了有效波段并且制定了支持向量机(SVM)框架下4种农作物识别的分类策略,分别在5∶5、6∶4、7∶3、8∶2、9∶1等5套随机样本分割方案下完成研究区域农作物的分类预测。在这20类分类精度中kappa系数均高于0.9609,总体精度高于0.9742;列向上5∶5分割方案的精度最高,8∶2的精度最低;横向上分类精度排序如下:SVM-RFE > SVM-RF > SVM-有红边波段 > SVM-无红边波段,该结果表明了红边指数和红边波段的参与显著地提高了作物的识别精度;(4) 由于水域等其他样本的缺少,SVM-RFE方法和SVM-RF方法的分类图像均存在少量错分现象。但从分类精度和图像细节展示上来看,SVM-RFE方法要优于SVM-RF方法,二者分类图像的交叉验证中kappa系数为0.8060,总体精度为0.8743。总之,高分六号红边特征在作物识别中表现优越,使得识别精度显著提高。后续研究者可开发更多与红边相关的植被指数,充分发挥红边特征在精准农业中的作用。
The application of red edge features, which are sensitive bands of vegetation, is a high-technology method for remote sensing to identify crops and realize precision agriculture. Multispectral GF-6 image of the study area in the northern region of Songnen Plain in Heilongjiang Province pioneers the use red edge bands in China. A total of 82859 crop samples of corn, soybean, and rice were used as research objects. The classification accuracy of crops was evaluated and the performance of red edge features in crop identification, such as red edge bands and vegetation index, was discussed from the following aspects. (1) Statistical characteristics of radiance values of crop samples initially showed that discrimination is better at Band 5-0.710 μm and Band 6-0.750 μm in the two red edge bands than findings of other GF-6 bands. (2) Traditional normalized (NDVI) and red-edge normalized difference vegetation indexes, namely, NDVI710 and NDVI750, are constructed. Results showed that the two indexes are more significant than the traditional NDVI in the classification of crop samples characterized using J-M distance. (3) Effective bands are screened using various methods, and classification strategies for the four types of crops are formulated using Support Vector Machine (SVM). Crop classification in the study area is completed using five sets of random sample segmentation schemes, namely, 5∶5, 6∶4, 7∶3, 8∶2, and 9∶1. Twenty types of classification accuracy demonstrated a kappa coefficient higher than 0.9609 and overall accuracy higher than 0.9742. The 5∶5 and 8∶2 segmentation schemes in the column direction exhibited the highest and lowest accuracy, respectively. The sorting accuracy in the horizontal direction demonstrated the following order: SVM-RFE > SVM-RF > SVM with red edge bands > SVM without red edge band, which also showed that the participation of red edge vegetation index and red edge band significantly improves the recognition precision of crops. (4) SVM-RFE and SVM-RF both obtained minimal misclassifications due to the lack of other samples, such as waters. However, SVM-RFE is superior to SVM-RF in terms of classification accuracy and image detail display with a kappa coefficient and overall accuracy of 0.8060 and 0.8743, respectively, in the cross-validation of two classified images. Hence, the red edge feature of GF-6 is superior in crop recognition with its significantly improved recognition accuracy. Subsequent investigations can focus on developing additional red edge-related vegetation indexes and optimize the role of red edge characteristics in precision agriculture.