下载中心
优秀审稿专家
优秀论文
相关链接
摘要
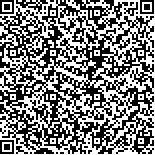
针对人工设计的中、低层特征难以实现复杂场景影像的高精度分类以及卷积神经网络依赖大量训练数据等问题,结合迁移学习与集成学习,提出了一种联合卷积神经网络与集成学习的遥感影像场景分类算法。首先基于迁移学习的思想,利用在自然影像数据集上训练好的多个深层卷积神经网络模型作为特征提取器,提取图像多个高度抽象的语义特征;然后构建由Logistic回归和支持向量机组成的Stacking集成模型,对同一图像的多个特征分别训练Logistic模型,将预测概率结果融合构建概率特征;最后利用支持向量机对概率特征训练和预测,得到场景影像的分类结果。利用UCMerced_LandUse和NWPU-RESISC 45两种不同规模的遥感影像数据集进行试验,即使在只有10%的数据作为训练样本情况下,本文方法能够分别达到90.74%和87.21%的分类精度。
Scene classification and recognition of remote sensing image is an important task for image interpretation. High-resolution remote sensing images have rich spatial texture features and semantic information, and their scene categories are diversified. As a result, images in the same category have a huge difference and some images in different categories become similar. which makes images difficult to be classified and recognized correctly. Therefore, choosing effective features and classification algorithms can improve classification performance. In this case, high-precision classification can only be achieved by selecting effective features and classifiers.Traditional scene classification algorithms adopt low-level or mid-level handcrafted features. These features have poor ability to represent high-level semantic information of images, which makes it difficult to achieve satisfactory results on massive complex scene images difficult. Deep learning, especially convolutional neural networks, has made great progress in computer vision. Compared with the methods using handcrafted features, deep learning is currently the most effective way for image classification. The application of a convolutional neural network to remote sensing image classification has achieved higher precision than methods using traditional handcrafted features. However, training a deep convolutional neural network that has too many parameters needs many labeled images, and the process of training is complicated and time-consuming. Generally, a deep convolutional neural network would not perform well with only a few images.A method for image classification using an ensemble convolutional neural network is proposed to improve the performance of convolutional neural networks. The method is composed of three main phases, namely, preprocessing, feature extraction, and ensemble learning. Firstly, the preprocessing stage includes geometry normalization, image intensity normalization, and image augmentation. Secondly, the feature extraction phase considers several deep convolutional neural networks, which have been well pre-trained on ImageNet, and are chosen to remove the last classification layer in the network and to extract different deep features of the same image. Thirdly, a stacking model is constructed in the ensemble learning phase. The stacking model consists of base and meta classifiers. The base classifier is composed of several logistic regression modes that are used to train different features extracted by deep convolutional neural networks. The meta classifier is a support vector machine. Finally, the probability distribution predicted by the base classifier is used to construct a new dataset that would be trained by the meta classifier.Experiments were conducted on two datasets named UCMerced_LandUse and NWPU-RESISC45 to verify the effectiveness of the proposed method. Compared with state-of-the-art methods, the proposed method performed better in overall accuracies. The proposed method could greatly improve performance and achieve overall accuracies of 90.74% and 87.21% on the two datasets, respectively, even with only 10% data used for training.With transfer learning, the features extracted by the deep convolutional neural networks are highly abstract and semantic, which have better ability in classification than other handcrafted features. Through feature fusion and model transferring, the advantages of different features and classification methods could be synthetically utilized. In this way, high classification accuracy could be achieved even with very little training data.