下载中心
优秀审稿专家
优秀论文
相关链接
摘要
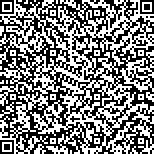
高光谱遥感影像具有光谱分辨率极高的特点,承载了大量可区分不同类型地物的诊断性光谱信息以及区分亚类相似地物之间细微差别的光谱信息,在目标探测领域具有独特的优势。与此同时,高光谱遥感影像也带来了数据维数高、邻近波段之间存在大量冗余信息的问题,高维度的数据结构往往使得高光谱影像异常目标类和背景类之间的可分性降低。为了缓解上述问题,本文提出了一种基于波段选择的协同表达高光谱异常探测算法。首先,使用最优聚类框架对高光谱波段进行选择,获得一组波段子集来表示原有的全部波段,使得高光谱影像异常目标类与背景类之间的可分性增强。然后使用协同表达对影像上的像元进行重建,由于异常目标类和背景类之间的可分性增强,对异常目标像元进行协同表达时将会得到更大的残差,异常目标像元的输出值增大,可以更好地实现异常目标和背景类的分离。本文使用了3组高光谱影像数据进行异常目标探测实验,实验结果表明,该方法与其他现有高光谱异常目标探测算法对比, 曲线下面积AUC(Area Under Curve)值更高,可以更好地实现异常目标与背景分离,能够更有效地对高光谱影像进行异常目标探测。
Hyperspectral images with high spectral resolution contain hundreds and even thousands of spectral bands and convey abundant spectral information to distinguish subtle spectral differences, especially between similar materials, thereby providing unique advantage for target detection. At the same time, hyperspectral images cause large number of bands and redundant information between adjacent bands. The high-dimensional data structure frequently reduces the separability between the anomaly and background classes of hyperspectral images. This study proposed a Band Selection-based Collaborative Representation (BSCR) method for hyperspectral anomaly detection to overcome these shortcomings. In BSCR, we first selected hyperspectral bands via an optimal clustering framework and obtained a set of representative bands. The separability between the anomaly and background classes enhanced. Then, we reconstructed each pixel in the image through collaborative representation. We obtained a large residual when reconstructing the anomaly pixel via collaborative representation and achieved a large output value for an anomaly pixel because of the enhanced separability between the anomaly and background classes, thereby improving the separation of the anomaly class from the background class. The proposed algorithm was tested on synthetic and real hyperspectral images. The experimental results of three hyperspectral images show that the proposed BSCR demonstrates outstanding detection performance in the receiver operating characteristic curve, area under the curve value, and separability map compared with other state-of-the-art detectors. BSCR has an improved discriminative ability to separate the target from the background. Compared with several traditional anomaly detection algorithms, BSCR enhances the separability between the target and background through band selection and can effectively separate the anomaly class from the background class in hyperspectral images. Algorithms, such as PCAroCRD, which also conduct collaborative representation after the dimensionality reduction of hyperspectral images, can remove certain anomaly pixels and make the background construction stable. However, the dimensionality reduction mode of such algorithms will change the original signal of the image, thereby making it smooth and increasing the difficulty to distinguish between anomaly and background classes. The dimensionality reduction method of the band selection in BSCR can enhance the separability between the target and background without changing the original signal of the image, thereby making it easy to distinguish when detecting anomaly pixels and enabling sufficient discriminative ability to separate the target from the background.