下载中心
优秀审稿专家
优秀论文
相关链接
摘要
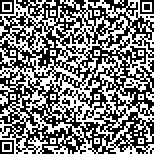
高分五号(GF-5)搭载的高光谱传感器兼顾宽覆盖和高分辨率的特性,但在实际应用中宽覆盖范围内各种地物类别的标注十分困难。当标记样本很少甚至没有标记样本时,遥感图像分类异常困难。此时,可以采用域适应方法,借助已标记的历史数据(源域)实现对未标记数据(目标域)的分类。本文提出了一种基于稀疏矩阵变换的关联对齐域适应分类算法。首先,利用稀疏矩阵变换估计源域和目标域的协方差矩阵;然后,运用协方差关联对齐方法估计源域到目标域的变换矩阵;接着,运用估计得到的变换矩阵将源域数据进行变换,使得其与目标域对齐;最后,在变换后的源域数据上建立分类器,实现对目标域数据的分类。本文的算法在两个真实的GF-5高光谱数据集上进行了验证。实验结果表明,本文算法要优于常用的子空间对齐算法和关联对齐算法。特别地,在黄河口GF-5数据上,本文算法比原始关联对齐方法的最近邻分类准确率提升了3.5%,支持向量机分类准确率提升了2.3%。
GF-5 is the first full-spectrum hyperspectral satellite used to achieve comprehensive observations of the atmosphere and land. The hyperspectral sensors on the GF-5 have high spectral resolution and wide coverage. However, labeling all the materials on these wide ranges is extremely difficult in practical applications. Hyperspectral classification is extremely difficult when the number of labeled samples is limited or no labeled sample is available. In this study, we aim to present an effective unsupervised domain adaptation technique that uses labeled pixels in the existing old domain(source domain)to classify the scenes with limited or no labeled pixels (target domain). Correlation alignment (CORAL) is a simple and effective domain adaptation method. However, the covariance computation in CORAL may be inaccurate in the case of limited training samples.We propose a new CORAL algorithm on the basis of a sparse matrix transform technique (CORAL-SMT) to solve this problem. The proposed method first uses the sparse matrix transform technique to estimate the covariance matrices of the source and target domains and then performs the CORAL between the estimated covariance matrices. The SMT method can obtain an accurate covariance estimator, which is constantly positive and definite. In the experiment, we compare the proposed CORAL-SMT with some classical domain adaptation methods, such as subspace alignment, principal component analysis, CORAL, transfer component analysis, geodesic flow kernel, and information the oretical learning. After domain adaptation, we use the nearest neighbor and support vector machine as classifiers to classify the unlabeled data in the target domain. Two GF-5 hyperspectral datasets, namely, Huanghekou and Yancheng datasets, are used to evaluate the performance of different methods. Experimental results demonstrate the effectiveness of the proposed method compared with subspace-based alignment methods and CORAL. The GF-5 data have good spectral discriminative ability. In the case of bias sampling, the performance of classifying the target samples on the basis of the source model is acceptable. The distribution difference between source and target domains is decreased, and the classification performance is intensively improved using the domain adaptation technique. The SMT technique can improve the covariance estimation,thereby benefiting the following domain adaptation.