下载中心
优秀审稿专家
优秀论文
相关链接
摘要
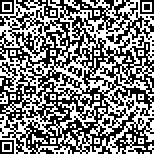
近年来,非负矩阵分解NMF(Nonnegative Matrix Factorization)由于其简单有效的特点,已被广泛应用于解混。由于传统的NMF只有单层结构,不能获取隐藏层的信息,其解混效果受到制约,为了研究影像的深度空谱特征,本文在深度NMF结构的基础上,提出了一种基于全变差和重加权稀疏约束的深度非负矩阵分解(RSDNMF-TV)算法。首先,使用深度NMF模型代替传统单层NMF模型,在预训练阶段进行逐层预训练,而在微调阶段减少分解误差。其次,由于丰度矩阵是稀疏的,本文在深度NMF模型中加入重加权稀疏正则化项,其权值则根据丰度矩阵自适应更新。最后,进一步引入全变差正则化项,以利用空间信息并促进丰度图的分段平滑性。论文采用梯度下降法推导出乘性迭代规则,为验证所提出的RSDNMF-TV算法的有效性,利用模拟数据集、Cuprite数据集以及高分五号数据集进行实验,并与其他经典方法作对比,结果发现本方法具有更好的解混效果,同时具有一定的去噪能力。
Hyperspectral unmixing, as a crucial preprocessing step for many hyperspectral applications, refers to the process of decomposing an image into a set of endmembers and corresponding abundance matrices. Nonnegative Matrix Factorization (NMF) has been widely utilized in hyperspectral unmixing because of its simplicity and effectiveness, whereas traditional NMF exits the local minimum problem. Various modified NMFs have been proposed to address such problem. Deep NMF has shown good performance in feature extraction using a multilayer NMF model. A novel deep NMF algorithm called reweighted sparsity regularized deep NMF with total variation (RSDNMF-TV) is proposed in this study by integrating the total variation and reweighted sparsity with deep layers. First, the deep NMF is obtained by extending the traditional single-layer NMF to the multilayer with pretraining and fine-tuning stages. The former stage pretrains all factors layer by layer, and the latter reduces the decomposition error. Second, a weighted sparse regularizer is integrated into the deep NMF model by sparing the abundance matrix, and its weights are adaptively updated in accordance with the abundance matrix. Finally, the total variation is introduced to improve the piecewise smoothness of abundance maps. In this study, gradient descent method is implemented for the multiplicative update. The experimental results obtained on simulated and real data sets confirm the effectiveness of the proposed method. For real data sets, we utilized the well-known AVIRIS Cuprite and GF-5 data sets. Six other algorithms, namely, total variation regularized reweighted sparse NMF, spatial group sparsity regularized NMF, minimum-volume CNMF, L1/2 sparsity CNMF, multilayer NMF, and VCA-FCLS, were used for comparison. The results on the three hyperspectral datasets show that the proposed unmixing method can outperform other algorithms. In summary, the proposed algorithm achieves improved performance with strong robustness and denoising ability, especially on images with low signal-to-noise ratio. However, RSDNMF-TV has several limitations, which are summarized as follows: (1) the deep NMF has higher computational complexity compared with the traditional NMF. (2) The proposed method only utilizes the prior knowledge of abundance and ignores the constraints on endmembers, such as spectral variability. (3) Parameter β is relevant to image’s spatial autocorrelation, and the adaptive selection of the parameter remains challenging. Future work will focus on solving these problems.