下载中心
优秀审稿专家
优秀论文
相关链接
摘要
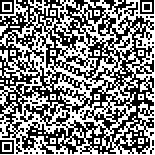
针对高光谱非线性混合模型中的共线性问题,提出了一种非监督的增强型非线性自编码网络方法ENAE(Enhanced Nonlinear Autoencoder)。通过结合自编码网络在挖掘数据内在结构、提取特征方面的优势,引入端元正则项减弱端元间的共线性效应,从而提高高光谱混合像元分解精度。ENAE方法的实现步骤主要包括两部分:一是网络结构初始化,二是非线性分解。网络结构初始化是确定编码器的节点数以及端元和丰度的初值;非线性分解则主要是实现损失函数的最小化。通过模拟数据、城市区域真实数据和高分五号卫星高光谱数据的实验,得到了相较于传统非线性分解方法更高的精度,证明了ENAE方法的鲁棒性。
The core of unmixing in hyperspectral images is to determine the mathematical form of the spectral mixing model in accordance with the radiative transmission characteristics of ground features and then obtain the endmember spectrum and abundance results. A nonlinear model is suitable for real mixed scenes because of the complexity of feature scenes. The accuracy of unmixing can be significantly improved by combining the advantages of autoencoder in internal structure data mining and feature extraction. However, this method cannot consider the collinearity of the model, resultingin overfittingand is sensitive to noise. This study proposed an unsupervised Enhanced Nonlinear AutoEncoder (ENAE) method. The introduction of endmember regularization reduces the collinearity between endmembers, thereby improving the accuracy of hyperspectral unmixing. The implementation steps of the ENAE method include two phases, where the first phaseis the initialization of the network structure, and the second phase is the nonlinear unmixing. The initialization phase determinesthe number of nodes of the encoder and initial value of endmember and abundance, and the nonlinear unmixing phase mainly realizesthe minimization of the loss function. The initialization of endmember and abundance aims to rapidly make the loss function converge. The objective function of the ENAE method includes the mean square error between the reconstructed and original images and endmember regularization. L2 regularization is used to constrain the weight of endmembers for enabling the ENAE to learn the nonlinear effect in the nonlinear mixing model. In the entire network iteration, the ENAE method is a self-learning process and does not require the participation of prior knowledge. Therefore, the ENAE method is an unsupervised nonlinear unmixing method. Experiments are conducted to validate the effectiveness of the proposed method. The experimental dataset includes simulation, urban real, and GF-5 satellite data. Three accuracy evaluation indices, namely, spectral angle distance, root mean square error of abundance, and image reconstruction error, are used to evaluate the effect of unmixing performance. Compared with the traditional nonlinear unmixing method, the deep learning method is superior in terms of endmember extraction and abundance estimation. The ENAE method can obtain high unmixing accuracy in the deep learning method, thereby provingthe effectiveness and robustness of the proposed method. The collinearity problem between endmembers is reduced by introducing the endmember regularization constraint in the autoencoder, thereby improving the accuracy of unmixing in hyperspectral images. In future work, we will introduce noise reduction, sparsity, and spatial information to improve the method and focus on obtaining the actual value of the pure ground spectrum to study the unmixing algorithm in hyperspectral images, which will be valuable in improving the application capabilities of hyperspectral remote sensing satellites in China. With the development of deep learning method interpretability research, exploring nonlinear unmixing methods withmany explanatory and physical meanings will be investigated forhyperspectral images withmixed pixel.