下载中心
优秀审稿专家
优秀论文
相关链接
摘要
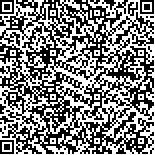
船舶作为海上的重要目标,实现对船舶自动识别有重要的意义。针对卫星图像中云雾、海岸背景等复杂海情对船舶识别带来的干扰,以及小目标船舶高漏检率问题,本文提出一种多尺度深度学习模型训练策略,在此基础上构建了一种船舶识别的深度学习网络,该网络可分为多尺度训练、特征提取、生成目标建议区域、船舶分类这4个部分。首先,采用多尺度的训练策略,将多尺度的船舶样本送入网络中进行训练,这样在训练样本中加入了大量小目标船舶的样本,使网络充分提取到小目标船舶的特征;其次,通过卷积神经网络对目标船舶进行特征自适应提取;然后,目标区域建议网络可依据卷积神经网络提取到的特征,在图像中找到感兴趣目标区域,即框定船舶的位置;最后,通过多个全连接层的组合,将高维特征映射到一个4元组中,再运用分类函数输出每一类船舶的概率值,概率值最大的则为该船舶的类别。同时为解决云雾遮挡和海岸背景的干扰,采用了一种负样本增强学习的方法,在样本数据集中加入了大量只含有云雾和海岸背景的图片,进行负样本扩充,增强网络模型对云雾及海岸背景的特征学习能力,以此解决复杂海情的影响。实验结果表明,所提方法有效解决了复杂海情条件下的船舶识别难,以及小目标船舶识别难的问题,实现了复杂海情条件下的船舶识别。同时,与现有成熟的深度学习目标识别算法相比,本文算法的精确度和召回率分别提升了6.98%和18.17%,所训练的模型具有良好的泛化能力和鲁棒性。
As an important target at sea, the realization of automatic recognition of ships is of great siguificance. The diversity of ship classes and complexity of marine environment exert higher requirements for fine-grained recognition of ships in satellite remote sensing images. Many domestic and foreign experts and scholars have carried out extensive research on ship recognition in remote sensing images. However, no universal method can recognize all kinds of ships. Most of the existing methods have strong specificity; poor portability, that is, these methods can only recognize certain classes of ship targets; and complex processing methods. This work proposes a multiscale deep learning model, which avoids complex modeling methods, such as template matching and background modeling. The network can adaptively determine the network parameters and realize the fine-grained recognition of ships by training large number of samples. First, this work uses negative sample enhancement learning to train the model, and fog and coastal backgrounds are sent as negative samples to the network for training to solve the influence of complex sea conditions, such as cloud–fog occlusion, and coastal background. At the same time, a multiscale sample training method is used in this paper in view of the problem that the size of some targets in the image is small and affects recognition accuracy. The images are compressed into multiple scales and sent to the network for training so that the network can fully learn the features of various ship sizes, thereby solving the difficulty of small target recognition. Second, the pre-trained ZF model is used for feature extraction, and the feature maps are sent to the region proposal network to generate proposal areas. Finally, the generated candidate areas are sent to the fully connected layer for ship fine-grained recognition. Experimental results show that the precision and recall of our method increased by 6.98% and 18.17% respectively, and the accuracy of ship recognition can reach 92.27% compared with Faster R-CNN. The method can guarantee real-time requirement based on high recognition accuracy and recognize ships under various conditions. The trained model can realize the fine-grained recognition of ships. The model not only solves the problems of cloud–fog occlusion to ships, but also the difficulty of small target recognition. The accuracy and real-time performance of the model meet the actual requirements and has strong robustness. However, the experimental results also show that the method used still has shortcomings. First, the constructed network structure has high complexity and excessive network overhead, which increase the processing time of ship target recognition; Secondly, the accuracy of the trained ship recognition model can still be improved. These two points are also the key tasks for the follow-up work.