下载中心
优秀审稿专家
优秀论文
相关链接
摘要
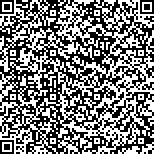
光学遥感图像船舶检测主要面临两个挑战:光学遥感图像背景复杂,船舶检测易受海浪、云雾及陆地建筑等多方面干扰;遥感图像分辨率低,船舶目标小,对于其分类与定位带来很大困难;针对上述问题,在FPN的基础上,提出一种融入显著性特征的卷积神经网络模型A-FPN(Attention-Based Feature Pyramid Networks )。首先,利用卷积提取图像特征金字塔;然后,利用顶层金字塔逐级构建显著特征层,抑制背景信息,通过金字塔顶层的细粒度特征提高浅层特征的表达能力,构建自上而下的多级显著特征映射结构;最后利用Softmax分类器进行多层级船舶检测。A-FPN模型利用显著性机制引导不同感受下的特征进行融合,提高了模型的分辨能力,对遥感图像处理领域具有重要应用价值。实验阶段,利用公开的遥感目标检测数据集NWPU VHR-10中的船舶样本进行测试,准确率为92.8%,表明A-FPN模型适用于遥感图像船舶检测。
Ship detection on spaceborne optical images is a challenging task that has attracted increasing attention because of its potential applications in many fields. Although some ship detection methods have been proposed in recent years, many obstacles still exist because of the large-scale and high complexity of optical remote sensing images. Identifying ships from interferences, such as the features of clouds, waves, and some land architectures that are similar to ships, is difficult. Therefore, an accurate and stable deep-learning based method is proposed in this work. The method involves three steps: First, the image feature pyramid is extracted using convolution to detect multiscale ship targets. Second, a multilevel attention feature mapping structure is constructed from top to bottom using the fine-grained features of the top layer from the pyramid to improve the expressive ability of shallow features. Finally, Softmax classifier is used for multilevel ship detection. The experimental results based on real remote sensing images are shot by “JL-1” satellite, Google satellite, and NWPU VHR-10. The result proves that the performance of our algorithm is better than the three other state-of-the-art methods. In addition, the network was cut while ensuring accuracy. The complexity of our algorithm is reduced, and its practicality is improved by experiments and analysis. This work proposes an attention-based method called A-FPN. However, unlike traditional algorithms, A-FPN has higher robustness and wider range of use. Furthermore, we effectively cut the network to reduce the complexity of the algorithm, thereby exhibiting the significance of our algorithm in practical applications.