下载中心
优秀审稿专家
优秀论文
相关链接
摘要
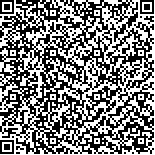
将关联向量机应用于高光谱影像分类, 实现高维空间中训练样本不足时分类器的精确建模。从稀疏贝叶\n斯理论出发, 分析关联向量机原理, 探讨一对多、一对一和两种直接的多分类方法。实验环节比较了各种多分类方\n法, 并从精度、稀疏性两方面将关联向量机与支持向量机等经典算法比较。实验结果表明, 两种直接的多分类方法\n内存占用大、效率低; 一对多精度最高, 但效率较低; 一对一计算效率最高, 精度与一对多近似。关联向量机精度\n不如支持向量机, 但解更稀疏, 测试样本较多时实时性好, 适合处理大场景高光谱影像的分类问题。
The relevance vector machine (RVM) is used to process the hyperspectral image in this paper to estimate the\nclassifiers precisely in the high dimensional space with limited training samples. The detail of RVM is firstly discussed based on\nthe sparse Bayesian theory. Then four multi-class strategies are analyzed, including One-vs-All (OAA), One-vs-One (OAO) and\ntwo direct multi-class strategies. In the experiments, the multi-class strategies are compared and RVM is further compared with\nseveral classical classifiers, including the support vector machine (SVM). The experiments show that two direct multi-class\nstrategies occupy too much memory space with low efficiency. OAA has the highest precision, but is low in efficiency. OAO is\nthe best in efficiency and the precision approximates to OAA. Compared with SVM, RVM is low in precision, but sparser than\nSVM. The sparse property is important when the test set is large, which makes RVM suitable for classifying the large-scale\nhyperspectral image.