下载中心
优秀审稿专家
优秀论文
相关链接
摘要
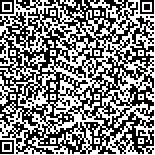
采用基于混合像元的结构分析方法和支持向量机(SVM)算法, 建立了高分辨率遥感数据(TM)向低分辨\n率遥感数据(MODIS)的尺度转换模型, 实现了由高分辨率遥感数据获得的NPP 向低分辨率遥感数据获得的NPP 的\n空间尺度转换。对低分辨率遥感数据(MODIS)估算的NPP 结果进行了尺度效应校正。结果表明: SVM 回归模型模\n拟出的尺度效应校正因子Rj_corrected 与1-F 中覆盖度草地之间的相关性较高, R2 达到0.81。尺度效应校正前的NPPMODIS\n与NPPTM 的相关性较低, R2 仅为0.69, RMSE 为3.47; 尺度效应校正后的NPPMODIS_corrected 与NPPTM 的相关性较高, R2\n达到0.84, RMSE 为1.87。因此, 经过尺度效应校正后的NPP 无论是在相关性还是在误差方面有了很大程度的提高。
Spatial scaling for net primary productivity (NPP) refers to the transferring process of establishing quantitative\ncorrelation between simulated NPP derived from data at different spatial resolutions. How to transfer NPP at one scale by the\nalgorithm with smaller error to at another is the urgent problem. Nonlinearity and effects from land cover type are two main\nproblems in NPP scaling. In this paper, the contextural approach based on mixed pixels and support vector machine (SVM)\nalgorithm are used to make the scaling model from the fine resolution (TM) to the coarse resolution (MODIS). Spatial scaling\nfrom NPP retrieved from fine resolution data to NPP derived from coarse resolution images is performed, and the correction of\nscale effect to NPP retrieved from coarse resolution data of MODIS is accomplished. The result shows that the correlation\nbetween Rj_corrected of the correction factor for scale effect and 1-Fmiddle density grassland estimated by SVM regression model is higher\n(R2=0.81). Before the correction for scale effect, the correlation between NPPMODIS and NPPTM is lower (R2=0.69; RMSE=3.47),\nwhile the correlation between NPPTM and corrected NPPMODIS_corrected is higher (R2=0.84; RMSE=1.87). Therefore, NPP corrected\nfor scale effect has been greatly improved in both correlation and error.