下载中心
优秀审稿专家
优秀论文
相关链接
摘要
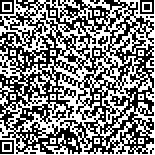
提出了一种利用Contourlet变换、Tsallis熵和改进粒子群优化的多源遥感图像匹配算法。在分别对参考图像和目标图像进行Contourlet分解的基础上, 以基于Tsallis熵的互信息量作为相似性度量准则, 利用改进的带极值扰动的简化粒子群优化算法对低分辨率的遥感图像进行匹配操作, 逐级上推, 最终实现全分辨率情况下多源遥感图像的匹配。实验结果表明, 与常用的遥感图像匹配算法相比, 该算法匹配精度高, 稳健性好, 且运算量大幅减少。
There are a lot of differences in multi-source remote sensing images from various sensors about the same scene. Maximization of mutual information can be used for the multi-source image matching, but the accuracy and efficiency of image matching need to be further improved. Therefore, an algorithm for multi-source remote sensing image matching was proposed in this paper, based on contourlet transform, Tsallis entropy based mutual information and improved particle swarm optimization. Firstly, the target image and reference image were decomposed to the low resolution image using contourlet transform, respectively. Then, a new image similarity measure criterion, the Tsallis entropy based mutual information, was used to achieve the global optimization. Meanwhile, a modified extremum disturbed and simple particle swarm optimization algorithm was applied to match the lowest resolution remote sensing images. Based on the preliminary result, the matching between the higher resolution images could be implemented stepwise up to the full resolution images. The experimental results show that, compared with those of other existing remote sensing image matching methods, the proposed algorithm has the high accuracy, strong robustness and requires much fewer operations.