下载中心
优秀审稿专家
优秀论文
相关链接
摘要
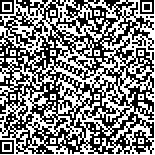
从空间数据场的角度出发, 提出了一种适用于空间聚类的场——凝聚场, 并给出了一种新的空间聚类度量指标(即凝聚力)。进而, 提出了一种基于场论的空间聚类算法(简称FTSC算法)。该算法根据凝聚力的矢量计算获取每个实体的邻近实体, 通过递归搜索的策略, 生成一系列不同的空间簇。通过模拟实验验证、经典算法比较和实际应用分析, 发现所提出的算法具有3个方面的优势: (1)不需要用户输入参数; (2)能够发现任意形状的空间簇;(3)能够很好适应空间数据分布不均匀的特性。
Spatial clustering is an important tool for spatial data mining and spatial analysis. It can be used to discover the spatial association rules and spatial outliers in spatial datasets. Currently most spatial clustering algorithms cannot obtain satisfied clustering results in the case that the spatial entities distribute in different densities, and therefore more input parameters are re-quired. To overcome these limitations, a novel data field for spatial clustering, called aggregation field, is first of all developed in this paper. Then a novel concept of aggregation force is utilized to measure the degree of aggregation among the entities. Further, a field-theory based spatial clustering algorithm (FTSC in abbreviation) is proposed. This algorithm does not involve the setting of input parameters, and a series of iterative strategies are implemented to obtain different clusters according to vari-ous spatial distributions. Indeed, the FTSC algorithm can adapt to the change of local densities among spatial entities. Finally, two experiments are designed to illustrate the advantages of the FTSC algorithm. The practical experiment indicates that FTSC algorithm can effectively discover local aggregation patterns. The comparative experiment is made to further demonstrate the FTSC algorithm superior than classic DBSCAN algorithm. The results of the two experiments show that the FTSC algorithm is very robust and suitable to discover the clusters with different shapes.