下载中心
优秀审稿专家
优秀论文
相关链接
摘要
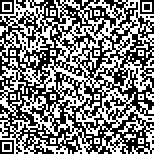
提出了一种基于粗集属性划分的遥感分类新方法, 构造了基于粗集的集成遥感分类器。该分类器利用粗集理论将输入的属性集合划分为多个约减, 利用这些约减构造多个训练子集。每个训练子集训练神经网分类器, 在决策时将多个单个分类器的结果进行投票选举。这种方法即减少了单个分类器的输入属性个数, 又避免了由于属性选取造成单一分类器在某些分类上的错误偏见。该分类器与神经网分类器方法, 以及属性选取与神经网结合方法进行了比较。结果表明RSEC无论在分类精度上, 还是在不同样本个数条件下的精度稳定程度上均有较好表现。
Supervised classification in remote sensing imagery is receiving increasing attention in current research. In order to improve the classification ability, a lot of spatial-features (e.g., texture information generated by GLCM) have been utilized. Unfortunately, too many features often cause classifier over-fit to a certain features’ character and lead to lower classification accuracy. The traditional feature selection algorithms have an unstable classification performance which depends on the number of training samples. This study presents a rough set based ensemble remote sensing image classifier (briefly denoted as RSEC). It partitions feature set into a lot of reducts, and constructs training subset by utilizing these reducts. Each training subset trains an artificial neural network (ANN) classifier; the decisions from all the base classifiers are combined with a voting strategy. This approach can reduce input features to a single classifier, and it can avoid bias caused by feature selection. The RSEC classifier has been compared with the direct ANN method and the traditional feature selection method. It can be seen from the result that RSEC has better classification accuracy and more stable than the others.