下载中心
优秀审稿专家
优秀论文
相关链接
摘要
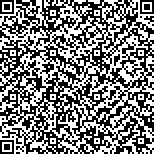
为提高图像处理效率, 探讨了面向对象分类的特征空间优化方法。以区域增长算法获得的对象为处理单元, 根据植被在IKONOS影像上的表征, 初步选择了6个形状、2个位置、17个光谱和6个纹理特征, 共计31个作为初始特征空间。首先根据每组中特征所代表的信息量和特征之间的相关性, 去掉与其他特征相关性强而方差较小的特征, 将特征空间维降到23;以识别城区植被为目标, 根据220个植被样本计算2—23维特征空间的类间J-M距离, 以最小J-M和平均J-M距离为依据选择最优特征空间, 将特征空间维降到14;最后利用类间离差矩阵代替协方差矩阵的K-L变换对特征空间进行压缩, 分组压缩后将维数降低到7, 而对整个特征空间压缩将维数降低到4。为验证特征空间优化对识别结果的影响, 采用CART分类方法对城市植被进行了识别。构建的决策树表明, 利用分组K-L变换后的特征空间比利用整体K-L变换获得的训练精度高12%;与K-L压缩前的特征空间获得的决策树相比, 结构复杂程度相当(前者包含14个结点, 后者包含12个结点), 训练精度仅低1%。分类结果也表明, 利用分组K-L变换的特征空间和不进行K-L变换的特征空间分类, 总精度和Kappa系数分别降低了1.5%和2.3%, 但是特征空间却压缩了50%, 提高了面向对象分类方法的处理效率。
Taking the identification on urban vegetation categories as an example, this study discussed feature set optimization methods to improve the efficiency of objected-oriented classification. Considering the characteristics of urban vegetations from IKONOS, 31 features were primarily selected, including 6 shape indices, 2 location features, 17 spectral and 6 texture features. Firstly, the features with low entropy and strong correlation with others were removed from the primary feature set, and the dimension of feature set was cut down to 23. From the point of identification on urban vegetations, the minimum and mean J-M distance were used to select the optimum feature set from 2 to 23 dimensions using 220 samples of vegetation patches, and the dimension of feature set was decreased to 14. K-L transformation was used to further decrease the dimension of feature set, in which deviation matrix between the target categories substituted the covariance matrix between different features, and the results showed that K-L transformation to the whole feature set compressed 70% of features and K-L transformation to the subgroup feature set compressed 50% of features, respectively. Comparing with the classification rules derived through CART, K-L transformation to subgroup feature set achieved the training accuracy 12% higher than the transformation to the whole feature set, and 1% lower than that without K-L transformation, respectively. The classification accuracy also showed that the total accuracy and Kappa coefficient using K-L transformation with subgroups decreased only 1.5% and 2.3%, but its feature set dimension decreased 50%.