下载中心
优秀审稿专家
优秀论文
相关链接
摘要
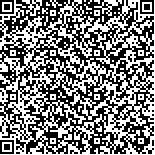
论文分析了时间序列遥感影像中土地利用/土地覆盖短期变化的特点及其时空异常特征, 认为和环境、物候等因素造成的影像变化相比, 由人为活动引起的土地利用/土地覆盖变化具有典型的时间和空间异常特征, 并提出了基于密度异常的土地利用短期变化检测方法。研究工作选取珠江口地区1—5月作物生长期间的3个时间序列Radarsat雷达影像进行试验, 在影像分割的基础上, 构建了基于对象的特征变化矢量, 并将密度异常检测算法(DBAD)扩展到变化矢量的N维特征空间上, 运用随机搜索策略确定检测参数, 对Radarsat时间序列变化矢量中的“小模式”事件进行了检测。检测结果认为, 密度异常检测算法检测的是变化矢量在特征空间的密度分布, 与变化矢量的强度和方向无关, 因此能在时间序列影像中分离出由典型的、正常的作物生长或农事活动引起的影像光谱或回波变化, 进而识别出由人为活动或突发事件导致的土地利用/土地覆盖变化, 这是通常的图像差值等方法难以做到的。进一步的抽样检测说明, 密度异常检测方法对新增建设用地的检测准确率最高(>88%);林地地表覆盖相对稳定, 检测误差也很低(8%);农用地和养殖水面的异常变化检测误差在11%—22%之间;较大的检测误差主要集中在建设用地、农用地和未利用地之间的转换(16%—25%);此外, 养殖水面的检测误差主要集中在河流沿岸及水面变化较大的养殖区域。影像分割结果特别是一些线状分割图斑以及混合地类图斑对误差也有一定的影响。
Although drastic land use change took place rapidly in very short interval in the Pearl River Delta of China, hardly any research has been done on this for lacking of available data. Located in the south of China, Pearl River Delta suffers from heavy cloud cover for more than half of the year. This makes real-time land use-land cover change (LUCC) monitoring almost impossible using optical remote sensing images. In this paper, the orbital highest resolution SAR (Synthetic Aperture Radar) data-Fine Mode Radarsat data is selected and three scenes of repeat-pass Radarsat images are used for short-term land use change detection. Short-term land use change caused by human activity is considered as spatial and temporal abnormal in time series images. And a Density-Based Anomaly Detection (DBAD) algorithm is designed to detect abnormally changed land parcels in time series Radarsat images. After that field survey data are used for validation. The result shows that DBAD gained better accuracy in comparison with object-based image regression method. Besides, DBAD exhibits greater capabilities in detecting under-constructed area and newly built up area (with error lower than 12%). While for built up area and some mixed used area, DBAD gained relatively lower accuracy (with error from 10% to 28.57%).