下载中心
优秀审稿专家
优秀论文
相关链接
摘要
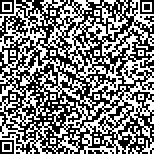
为解决合成孔径雷达(SAR)图像存在大量混合像元的问题,针对传统ICA不能有效解决混合像元分解这一缺陷,提出一种新的独立成分分析算法--有监督学习ICA算法(SL-ICA).其目标函数是在原ICA负熵目标函数基础上增加监督学习的约束条件项,进而在同一目标函数内实现负熵和约束条件的统一,在最大化负熵的同时也最小化了约束条件的误差,此外,采用一种新的双梯度下降法优化迭代,提高计算速度.并以人工模拟SAR图像和北京地区ENVISAT-ASAR作为数据源进行实验,实验结果明显优于主成分分析方法(PCA)的分解结果.
Forresolving theproblem thatthere are lotsofmixed pixels in the Synthetic Aperture Radar(SAR) images, againstthe flaw that the traditional Independent Component Analysis(ICA) can not solve the decomposition ofmixed pixels effectively, we propose a new algorithm: Supervised Learning ICA algorithm(SL-ICA). Adding supervised learning restrictive conditions to the negentropy objective function, we implementnegentropy and restrictive conditions in a unified objective function, which minimizes the errorwhilemaximizing the negentropy. At the same time, we optimize the objective function using a new dual-gradientdescent algorithm iteratively, which accelerates the computing speed. By testing SL-ICA and PrincipalComponentAnalysis (PCA).on artificial simulated SAR images and ENVISAT-ASAR (Advanced Synthetic Aperture Radar) images ofBeijing, the results show that SL-ICA can getmore precise results than the PCA.