下载中心
优秀审稿专家
优秀论文
相关链接
摘要
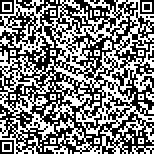
高光谱遥感技术,将反映目标辐射特性的光谱信息与反映目标空间位置关系的图像信息有机地结合在一起.高光谱影像具有丰富的光谱信息,较全色、多光谱影像能够更好的进行地面目标的分类识别.在介绍核Fisher判别分析算法的基础上,选用径向基核函数,使用一对一或一对余构造多类构造法,并利用交叉验证网格搜索法优化核函数参数,构建了快速稳定的多类核Fisher判别分析分类器.通过OMIS和AVIRIS影像的分类实验,表明了核Fisher判别分析与支持向量机的分类精度相当,但是所需的训练时间较短.
关键词:
高光谱遥感 分类 核Fisher判别分析 核函数The hyperspectral remote sensing technology, which appeared early in 1980s, combines the radiation informationwhich relates to the targets attribute, and the space information which relates to the targets position and shape,completing the information continuum of optics RS mi age from panchromatic mi age to hyperspectral via multi-spectral mi age. The spectrum information,which is rich in the hyperspectral mi age, comparedwith panchromatic remote sensing mi age andmultispectral remote sensing mi age, can be used to classify the ground targetbetter. Ithas become an mi portant technique ofmap cartography, vegetation investigation, ocean remote sensing, agriculture remote sensing,atmosphere research, environmentmonitoring andmilitary information acquiring.As SupportVectorMachine (SVM) was applied tomachine learning fields successfully in recent years, the classic linear pattern analysis algorithmswhich was called the 3rd revolution of pattern analysis algorithms, can cope with the nonlinear problem. Some references applied the kernelmethods to linearFisherDiscrmi inantAnalysis (FDA), and put forwardKernelFisherDiscrmi inantAnalysis (KFDA).Firstly, this paper introduced the classification method based on the kernel fisher discrmi inant analysis.For the binary problem, the ami ofFDA is to find out the linear projection (projection axes) on which the intra-class scatter matrices of the training samples aremaxmi ized and scattermatrices of inter-class areminmi ized. ForKFDA,the inputted data ismapped into a high dmi ensional feature space by a nonlinearmapping, while linear FDA in the feature spacewill be performed.Secondly,we researched on the selectionmethods of the kernel function and itsparameter,and studied on themulti-classes classificationmethods, and then applied them tohyperspectral remote sensing classification. We use decomposition\nmethods ofmulti-class classifier andmethod ofparameter selection using cross-validating grid search to build an effective and robustKFDA classifier.Finally, we carried outthe hyperspectral mi age classification expermi entsbased onKFDA and some othercomparative expermi ents. Some conclusions can be drew as follows.Using the kernelmapping, the KFDA expermi ent on PHI and AVIRIS mi age demonstrates that the KFDA is less affected by the dmi ension of input sample, and can avoid theHughes phenomena effectively. The results show that ithas more comparable classification accuracy than supportvectormachine classifier.There is no need to compute the complicated quadratic optmi izing problem in training KFDA classifier as SVM classifier does, so this algorithm is not very complicated and costs less tmi e. Especially in the one-against-rest decomposition, comparingwith the SVM, KFDA ismuch faster.The capability of KFDA classifier is affected a lot by kernel function and its parameters, and a fine recognition precision can only be obtainedwhen the kernel function s parameters are appropriate. The stability ofclassification can be effectively mi proved by parameter selection via cross-validate grid searchmethod.