下载中心
优秀审稿专家
优秀论文
相关链接
摘要
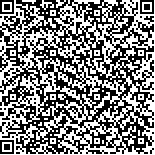
智能式遥感分类是遥感研究的新热点.提出了一种基于蚁群智能规则挖掘(ant-miner)的遥感影像分类新方法.遥感数据各波段之间存在较强的相关性,这种相关性往往会导致分类产生误差.而ant-miner算法中的信息素是基于规则整体性能的,信息素的动态更新能有效地处理相关性较强的数据,所提供的正反馈信息能纠正启发式函数缺陷所造成的错误.因此,蚁群智能算法应用于遥感分类具有一定的优势.将该方法用于广州市地区的遥感影像,取得了较好的分类结果.并与See5.0决策树方法及最大似然方法(MLH)进行了对比研究,实验结果表明,蚁群智能算法分类精度比后两者的分类精度更高.
This paper presents a bottom-up approach to mi prove the classification performance for remote sensing applications. Top-down approaches, such as statistical classifiers, have inherited lmi itations in dealingwith complicated relationships in classification. Forexample, data correlation between bandsofremote sensing mi ageryhas caused problems in generating satisfactory classification with statisticalmethods. In this paper, ant colony optmi ization (ACO) based on swarm intelligence is used to mi prove classification performance. Actually, ACO is a complex multi-agent system, in which agentswith smi ple intelligence can complete complex tasks through cooperation such as classification problems.Ants guide their route selection based on pheromone, which is accumulated from the collectivemovements of individual ants. In thisway, an ant learns from the pastexperience ofothers. Ant-Miner is different from decision tree approaches.The entropymeasure is a local heuristicmeasure, which considers only one attribute at a tmi e, and so it is sensitive to attribute correlation problems. Whereas inAnt-Miner, pheromone updating tends to cope betterwith attribute correlation,since pheromone updating is directly based on the performance of the rule as awhole. Thus, Ant-Miner should have great potential in mi proving remote sensing classification. In this study, an Ant-Miner program for discovering classification rules is developed for the classification of remote sensing mi ages. In theAnt-Miner program, the route search by an ant colony is to find the best links between attribute nodes and class nodes. An attribute node corresponds to a band value of remote sensing mi ages. An attribute node can only be selected once andmustbe associated with a class node. Each route corresponds to a classification rule, and discovering a classification rule can be regarded as searching for an optmi al route.To enableACO to effectively classify remote sensing mi agery of very large data sets, original band values are sliced into a number of intervals by using a discretization technique. The ACO method is more explicit and comprehensible than mathematical equations. Our study in Guangzhou city indicates that the ant colony-based classifier yields better accuracy than conventionalmaxmi um likelihood classifiers and decision tree classifiers. The overall accuracy of theACO method is 88.6%, with aKappa coefficientof0.861. The decision treemethod has an accuracy of85.4% and aKappa coefficientof 0.822. Themaxmi um likelihoodmethod has an accuracy of83. 3% and aKappa coefficient of0. 796. The results clearly support the conclusion that themethod explored in thispapercan bemore effective than conventional classificationmethods.