下载中心
优秀审稿专家
优秀论文
相关链接
摘要
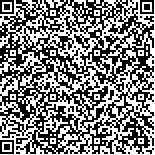
遥感图像分割是对遥感图像进行处理的最为关键的一步.马尔科夫随机场模型作为先验模型,在图像分割领域已经得到了广泛的应用,实践证明该模型有助于提高图像分割的效果.但是由于环境和传感器的影响,遥感图像具有灰度变化大、纹理复杂及边界模糊等特点,经典的马尔科夫随机场模型在遥感图像分割中的分割效果通常并不理想.本文针对遥感图像分割中某些像素分类的不确定性,建立了模糊马尔可夫随机场模型(FMRF).该模型结合分割问题中的随机性与模糊性,更合理地获取了图像的先验知识,较好地符合了遥感图像的特点,因而使得图像分割过程中使用先验知识更为准确.同时算法针对遥感图像的特点,结合了图像的灰度特征和纹理特征,从而使其能更准确地区分图像中的不同类.为使两种特征能够很好地结合,本文采用了贝叶斯分割方法,使用权值对图像特征进行权衡.同时本文采用最大期望算法(EM)对不完整的数据进行估计,应用模拟退火算法(SA)获得全局最优解,从而实现了无监督分割.实验证明,对于SAR图像,该方法较经典的马尔可夫随机场(MRF)算法和模糊C-均值(FCM)算法更好地处理了边缘的混叠,明显减少了斑点噪声,使分割结果更加准确.
Remotesensing mi age segmentation is the process to divide the mi age into regionswith different features and extract the object through segmentation,whichwill probably be used into the next processing step. Remotesensing mi age segmentatian is an mi portant step from mi age processing to mi age analysis.In recentyears, segmentation of remote sensing mi ages plays themost mi portant role in the interpretation of remote sensing mi ages, but there is no reliable model to guide the remotesensing mi age segmentation. Markov Random Field (MRF) has been extensively applied in segmentation of mi ages as priori probabilitymode,l and in fact, thismethod can be applied to mi prove the resultofsegmentation of mi ages. Influenced by environmentand the sensor, remote sensing mi a-geswith complex texture, large brightness range and vague bridge boundary, can notbe apt to use the standardMRF, and the resultofsegmentation ofremote sensing mi agesusing standardMRF isnotsatisfactory.Therefore in remote sensing mi-age segmentation, the algorithm using classicMRF is often inefficient.Ami ing at ambiguity in the segmentation of remote sensing mi ages, theFuzzy MarkowRandom Field (FMRF) is put forward in this paper. Framework in segmentation algorithm based on FMRF is proposed. Themethod solves problems re-sulting from randomicity and fuzzability and gets the priori probabilitymodel reasonably, smi ultaneously, adds the gray feature and the texture feature into the segmentationmodel in order to getmore accurate result. In the past,the techniques in fuzzy segmentation focused on the gray feature of mi age, so there are obviousdefectswith these techniques inmulti-level classification. Under theMaxmi um aPosterioriPrinciple(MAP)MRF framework, we extractgray and texture features. We developemodels respectivelywith their features. The expermi ents show that it is efficient tomulti-level mi ages.Finally, there are less parameters used in thismethod, however, segmentation needs ability to learn parameters and to realize unsupervised mi age segmentation. We apply the smi ulated annealing (SA) and expectation-maxmi ization (EM) algorithm to estmi ate unknown parameters and get the globaloptmi al solution. The segmentation expermi ents ofSAR mi a-ges demonstrate that the proposed algorithm ismore efficient to distinguish interlaced edges and restrain the speckle noise than the algorithm of the FuzzyC-Mean FCM and standardMRF.We apply algorithms that arementioned in this paper to SAR mi ages segmentation withVC++ 6. 0,and compare indicators of segmentation by different algorithms.