下载中心
优秀审稿专家
优秀论文
相关链接
摘要
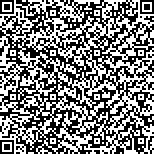
作者 | 单位 | |
王鹏伟 | 中国科学技术大学 电子工程与信息科学系 安徽 合肥 230027 | wangpw@mail.ustc.edu.cn |
李滔 | 中国科学技术大学 自动化系 安徽 合肥 230027 | |
吴秀清 | 中国科学技术大学 电子工程与信息科学系 安徽 合肥 230027 |
提出了一种基于SVM后验概率的MRF分割方法,将支持向量机的后验概率应用于Markov随机场方法中,通过贝叶斯公式将对样本条件概率的估计转换为后验概率估计,再通过对SVM决策函数输出的映射来产生后验概率,并将SVM估计的后验概率信息带入MRF模型实现分割,从而完成了一种新的Markov随机场模型的分割方法.实验结果表明,采用此方法分割纹理图像可以获得较好的分割结果.
A novel segmentationmethod based onMarkovRandom Field (MRF) and SupportVectorMachine (SVM) posteriori probability is proposed in the paper. As a rule, mi age segmentation usingMRFmodel has two steps. Firstly,distribution of conditional probability of pixel characteristic is obtained by the parameter estmi ate for probability density and thenmaxmi um a posteriori(MAP) principle is always used to gain the optmi um estmi ate of class labe.l In practice,the hypothesis ofGaussdistributionmodel is always adopted, but it isnot themodel fit forany mi ages, for example, SAR miages often fit to amodel of Rayleigh distribution and especially some texture mi ages, it is very difficult to deduce an accurate distributionmode.l In order to solve the twomajor problemswhich are the complexity of parameter estmi ate in using the distribution of conditional probability and the difficulty ofdeducing an accurate distribution in theoreticalway,the new segmentation approach based onMRF and SVM posteriori probability is proposed.SupportVectorMachine is a setof related supervised learningmethod,it is a classification technique based on the structural riskminmi ization principle and itmaps inputvectors to a higherdmi ensionalspacewheremaxmi alseparating two parallelhyperplanes are constructed. An assumption ismade that the larger themargin ordistance between these parallel hyperplanes the better the generalisation error of the classifier will be. However, in the pattern recognition practice,people need soft decision, that is to say, not only gain class label which the sample belongs to, but also obtain the membership degree ofsample in each class labe,l that is posterioriprobability ofsample. The new segmentation algorithm proposed by the paper follows three steps. Firstly, the paper adopts the Platt smethod to obtain the posteriori probability bymapping the outputofSVM decision-function after training. Secondly,it converts the conditional probability estmi ate into posterioriprobability estmi ate in termsofBayes formula, and then proposes a new segmentationmethodwhich depends onMRFmodelbased on posteriori probability. Finally, itbrings the information of posteriori estmi ate intoMRFmode,l thus the posteriori probability based on SVM is combinedwithMRF in the application of mi age segmentation. Two experi-ments has been conducted, one is that the paper selects twelve texture mi ages from Brodatz standard texture database to make up somemerged texture mi ages. The number of training samples in each texture class is 30 and eight features are used to characterize each texture sample. The resultsofthree group segmentation expermi ents allshow thatthe newmethod appears preferable toGaussianMRFmethod. The otherexpermi ent is that the synthesis texture mi age is composed by SAR texture mi ages, SAR mi agesdo not fit forGaussdistribution, as a result, GaussianMRFmethod results in highmisclassi-fication rate and low robustness. On the contrary, the proposed algorithm depends on the information of posteriori probability estmi ate based on SVMwithout the hypothesis of sample conditionalprobability, so itachieves a higher levelof robustness and segmentation results demonstrating its efficiency.