下载中心
优秀审稿专家
优秀论文
相关链接
摘要
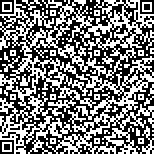
本研究利用水稻冠层高光谱数据,模拟NOAA-AVHRR,Terra-MODIS和Landsat-TM的可见光波段反射率数据,计算各传感器的多种植被指数(NDVI,RVI,EVI,GNDVI,GRVI和Red-edge RVI),比较植被指数模型对水稻LAI的估测精度,分析不同植被指数对LAI变化的敏感性.相对于红波段植被指数,红边比值植被指数(Red-edge RVI)和绿波段指数GRVI与LAI有更好的线性相关关系,而GNDVI和LAI呈现更好的对数相关关系.MODIS的Red-edge RVI指数不仅模型拟合的精度最高,还有独立数据验证的估测精度也最高,而且它的验证精度较拟合精度下降幅度最小;其次是绿波段构建的GNDVI和GRVI植被指数的估测精度,再次是NDVI和EVI的估测精度,而RVI的估测精度最差.敏感性分析发现,13个植被指数对水稻LAI的估测能力都随着LAI的增加而下降,但归一化类植被指数和比值类植被指数对LAI变化反应的差异明显,归一化类植被指数在LAI较低时(LAI<1.5)对LAI变化的反应开始非常敏感,但迅速下降,而比值类植被指数在LAI较低时,明显小于归一化类植被指数,之后随着LAI的增大(LAI>1.5)比值类植被指数对LAI的变化敏感性,则明显高于归一化类植被指数.Red-edge RVI和绿波段指数GRVI和LAI不仅表现了很好的线性相关关系,而且在LAI大于2.9左右保持较高的敏感性.
关键词:
植被指数 叶面积指数 估测精度 敏感性 水稻 传感器 模拟 不同植被 指数对 水稻叶面积指数 估测 拟合精度 敏感性分析 Rice LAI Sensors Different Vegetation Indices Simulated Regression Models 表现 变化反应 差异明显 归一化 能力 发现In this study,vegetation indices(VI) were firstly calculated from the simulated band reflectance spectra of NOAA11-AVHRR,AQUA-MODIS and LANDSAT5-TM using rice canopy hyperspectral reflectance spectra,which were acquired in 2002 and 2004.Regression models for estimating LAI were fitted by that of 2002 and validated by the VIs data sets of 2004.Estimating accuracies between models were compared and analysis of VIs' sensitivity to changes in LAI was performed.Due to differences in spectral response function,the simulated red band reflectance of AVHRR was higher,whereas near infrared reflectance was lower than that of TM or MODIS for identical rice canopy.Compared with the models of VIs calculated from the red band reflectances,LAI showed closer linear relationship in models of ratio VIs from the red-edge or green band reflectances and closer exponential relationship in models of normalized VIs from the green band reflectances.Among others,the model using Red-edge Ratio Vegetation Index(Red-edge RVI) showed the highest estimating accuracy not only for calibration but also the highest one for external validation,furthermore the decrease in estimating accuracy between calibration and external validation was lowest.The models of Green Normalized Difference Vegetation Index(GNDVI) and Green Ratio Vegetation Index(GRVI) held the second highest position of estimating accuracies for external validation,the models of normalized difference vegetation index(NDVI) and enhanced vegetation index(EVI) were the third highest and those of RVI were the worst.Although AVHRR bands have broader ranges of spectral response than TM and MODIS,their NDVI and RVI models exhibited higher estimating accuracies for rice LAI in this study.The sensitivities of all indices decreased with the increase of LAI values,but difference of sensitivities between normalized VIs and ratio VIs was significant.The normalized VIs exhibited much higher sensitivities with rapidly decreased rate than the ratio VIs at LA1 values of less than 1.2,while the ratio VIs did higher sensitivities than the normalized VIs at LA1 values of more than 1.2.Red-edge RVI and GRVI exhibited not only higher linearity relationship with LAI but also higher sensitivities at LAI values of more than 2.8.When comparison of model fitness between linear and nonlinear regression models is made,it should be done not by directly comparing the coefficients of determination of regression models but the coefficients of determination of linearity relationship between the predicted and the observed.