下载中心
优秀审稿专家
优秀论文
相关链接
摘要
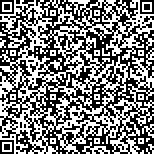
准确的遥感农作物类型识别和种植面积统计,不仅仅取决于不同分类方法的选择,同时还要看输入分类器用以学习的训练样本数据,训练样本对分类精度的影响比分类技术本身对测量精度的影响还要大。训练样本对测量精度的影响包含样本的质量和数量两个方面。为了探讨训练样本对农作物种植面积测量精度的影响,本文以小麦为例,选择典型试验区,利用较常用的TM遥感影像,结合高分辨率SPOT数据和野外GPS数据,在构建标准训练样本和检验样本数据集的基础上,在不同训练样本量下,分别对光谱角制图、平行六面体、马氏距离、最小距离、最大似然和支持向量机6种方法进行了多次试验,并对测量结果进行了对比分析。研究结果表明:①不同样本量下6种方法10次测量的结果存在不同程度的波动,而且地块越破碎的地区波动越大,但这种波动随样本量的增加会减少,说明目前在小麦面积测量中,用单次分类的结果作为最终的提取结果的做法存在一定的随机误差,在实际的工作中,应尽可能多的获取训练样本,以提高小麦面积测量的稳定性,同时利用多次测量结果求均值的方式,来消减或者抵消测量的随机误差;②相同样本量下,各种方法测量出的全区和破碎区的小麦像元个数(总量)不一致,种植结构复杂且破碎的地区,各种方法测量出的小麦面积总量差异会更大,说明不同方法对同一地区小麦的识别能力是不同的,同种方法对于不同地区小麦的识别能力也是不一样的,但是通过分析小麦识别的产品精度和用户精度,可以判断出哪个结果最接近于真实总量。
Exact agricultural crops identification and planting areameasure depend on notonly classifiers but also training samples mi ported into classifiers. The effect of training samples, including sample quality and quantity, is greater than that of classifiers on measure accuracy. In order to probe into the effect of training sample on agriculturalplanting areameasure, a representative wheatsowing sectionwas taken as an example. A standard sample setwas constructed based the popular TM miage, SPOT-5 mi agewith high resolution and field data from GPS. Then training sampleswith same features and differentnumberswere respectively input into six different classifiers to classify miage and identify wheat, which are spectral angle mapper, parallelepiped,mahalanobis distance, minmi um distance, maxmi um likelihood and support vector machine. Moreover, the expermi entationwas repeated ten tmi es under different sample numbers. The results indicate:①Tenmeasure results fluctuate from sixmethodsunderdifferent training sample numbers. The fluctuation is reducingwith the increasing of training sample numbers, which is greater in more fragmentized plot. There are some random errors in the current common used method of wheat planting area measure, which always uses one tmi e\nclassification result as the finalvalue. In the task ofagriculture planting areamensure, samples should be used asmany aspossible in classification to advance the resultstability and the average of lotsofclassification results is advised to counteract the random errors.②Thewheatpixelnumbers(region whole area)differ each other among sixmethods under the same training numbers. And the diversity is larger in area with complex plant structure and fragmentized plots. Different classifier has different response to the same area, while same classifier has different response to different area. But it can be judge and verdict, which result is nearer to the true value, through analyzing the product accuracy and user accuracy ofwheat.