下载中心
优秀审稿专家
优秀论文
相关链接
摘要
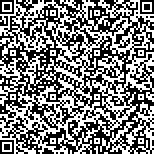
遥感数据具有在不同空间、光谱和时间尺度上获取地表测量信息的能力,使其成为获取土地覆盖信息的一个主要数据源。影像分类即把卫星影像上的相关像元划分给某类已知的土地覆盖类型的过程。支撑向量机(SVMs)是一种土地覆盖分类的新技术。三种常用的SVMs是:基于线性和多项式的SVM以及具有高斯核函数的SVM分类器,分类能否成功地应用有赖于其各自选择的最佳参数。但是海量的遥感数据使得这些参数的确定速度十分缓慢。本文研究了一种新的基于最优化遥感数据压缩技术的SVM分类方法。研究显示用于获取SVM参数的数据量能够在不影响土地覆盖的分类精度的前提下进行压缩。数据压缩成功的应用于多项式和高斯核函数的SVM分类,而线性SVM的分类精度却非常低。
The ability of remote sensing data to acquire measurements of land surfaces at different spatial,spectral and temporal scales renders it a major source of land cover information.The process of relating pixels in a satellite image to known land cover is referred to as image classification.Support Vector Machines(SVMs) represent one of the new generation land cover classification techniques.There are three commonly used SVMs namely:linear,polynomial and radial basis function(Gaussian) SVM classifiers,whose successful deployment is dependant on the selection of respective optimum parameters.The voluminous nature of remote sensing data renders the identification of these parameters slow and tedious.In this paper,a new data reduction technique that optimizes remote sensing data for SVM classification is proposed.This research shows that the quantity of data needed to derive the SVM parameters can be reduced without adversely affecting the land cover classification accuracy.Data reduction was successfully applied to polynomial and radial basis function(RBF) SVM classification.Linear SVM classification results however,resulted in significantly poorer classification accuracies.