下载中心
优秀审稿专家
优秀论文
相关链接
摘要
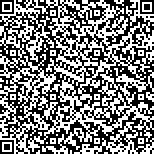
遥感图像分类是一项十分重要而且复杂的问题。传统的图像分类方法多数是基于贝叶斯主观概率理论的图像分类方法,由于其在解决不确定性问题上存在诸多缺陷,近年来,将数学的证据理论应用于遥感图像分类已成为新的发展趋势。本文首先介绍了证据理论与贝叶斯主观概率理论对于解决不确定性问题的差异,证据理论的主要定义和算法。文中还重点介绍了证据理论用于模式识别的支持度函数,并将支持度应用于图像分类,得到像元级按大类(如土地利用的城镇用地、耕地、林地和水体)划分的支持度表面,然后进行硬分类叠加,得到初次分类结果,再进行精度评估。如果不满足精度要求,再对各类支持度小于某域值的像元进行二次分类,如此下去,直到达到分类所要求的精度。该方法的主要优点是可以进行分类后的再分类,且精度非常高,而贝叶斯分类不可以进行分类后的再分类,只能重新训练样本进行整体分类,效率低,精度也难提高。该方法理论可靠,实用性强,易操作,有研究潜力。
Remote sensing image classification is an important and complex problem.Conventional remote sensing image classification methods are mostly based on Bayes' subjective probability theory.Because there are many defects,a new tendency is that the mathermatical theory of evidence has been applied to remote sensing image classification.At first,this paper introduces differences between Dempster-Shafer's evidence theory and Bayes' subjective probability theory and main definitions and algorithms on D-S evidence theory.Especially degree of belief,degree of plausibility and degree of support are the bridges that D-S evidence theory is used in other fields.It emphatically introduced Support function that D-S evidence theory is used on pattern recognition,and degree of support is applied to classification.We acquire degree of support surfaces according to large classes,such as urban land,farmland,forest land,and water,then use "hard classification" to gain initial result.If initial classification accuracy is fitted to the acquirement,reclassification for degree of support surfaces of less than threshold is conducted until final classification result reaches accuracy.Main advantages of this method are that it can perform reclassification after classification and its classification accuracy is very high.This method has dependable theory,intensive application,easy operation and research potential.