下载中心
优秀审稿专家
优秀论文
相关链接
摘要
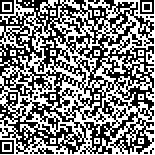
提出了一种像元形状指数及基于形状和光谱特征融合的高(空间)分辨率遥感影像分类方法。形状和光谱是遥感影像纹理的具体表现形式,尤其在高分辨率影像中地物细节得到充分表达,相邻像元的关系及其共同表征的形状特性成为分类的重要因素。本文用像元及其邻域的关系来描述其空间结构,同时为了更全面地利用影像特征,提出了基于支持向量机的形状和光谱融合分类方法。实验证明,该方法计算简便且能有效表达高分辨率影像的地物特征,提高分类精度。
Classification of multispectral data with high spatial resolution is investigated.High spatial resolution remotely sensed(HSRRS) images with multispectral bands such as QuickBird and IKONOS provide a large amount of information,thus opening up avenues for new remote sensing applications.However,their availability poses challenges to image classification.Due to the complex spatial arrangement and spectral heterogeneity even within the same class,conventional spectral classification methods are grossly inadequate for HSRRS imagery.In order to overcome this inadequacy,spectral features must evidently be complemented by one or the other means.Shape is an important feature of high spatial resolution remotely sensed(HSRRS) imagery,and it is the manifestation of textures on such imagery.In this paper,a spatial feature index,pixel shape index(PSI),is proposed in order to describe the shape feature in a local area surrounding a pixel.PSI is a pixel based feature,which measures the gray similarity distance in every direction.As merely the shape feature is inadequate for classification of HSRRS imagery,the spectral bands are added to the input vectors of our classifier.And then a fast fusion algorithm which integrates both shape and spectral features using the support vector machine(SVM) has been developed to interpret the complex input vectors.To test the effectiveness of PSI,some spatial features extracted by wavelet transform(WT),gray level co-occurrence matrix(GLCM) are utilized for comparison.Experimental results demonstrate that PSI is capable of describing the shape features effectively and leads to more accurate classifications than other methods,meanwhile the classification algorithm fusing spectral and shape features by SVM is feasible.It is also found that spectral and shape features can complement each other and their integration can result in enhanced accuracy.