下载中心
优秀审稿专家
优秀论文
相关链接
摘要
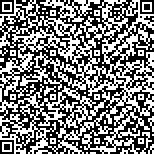
本论文尝试讨论两个主题:主题一为利用主成分分析PCA方法应用于像元阶层资料融合技术的研究。主题二为应用Dempster-Shafer evidence theory方法于特征阶层数据融合技术的研究。在第一个主题中,由于合成孔径雷达的数据具有全偏极特性,在此选取了对植被较为敏感的HV极化合成孔径雷达数据,与具有光谱特性的光学SPOT数据做数据融合处理以利接下来的地物分类。首先,本研究利用小波转换技术来滤除合成孔径雷达斑驳噪声,在接下来融合步骤中,主成分分析出来的第一部分(PCI)是用做完滤除噪声后的合成孔径雷达取代,在数据融合后,进行地物分类是采用最大似然法来分类融合影像。在第二个主题中,利用全偏极雷达数据的极化特性结合SPOT数据的光谱特性,其主要目的是为了增加分类的精确度。首先使用李式滤波器滤除全偏极雷达数据噪声,接下来同样是使用采用最大似然法来分类融合影像,(不同的在于全偏极雷达影像使用Wishart几率分布,在光学影像采用multivariate Gaussian几率分布)将每个类别中每个像元属于某个类别的几率值计算出来,再利用Dempster-Shafer evidence theory来结合这些类别的机率值。最后产生出一张新的分类影像。实验的结果显示分类的精确度比较于未融合的资料都有明显提升的效果,也证明了此两个数据融合方法对于不同数据特性的融合都是很成功的。
There are two main topics to be discuss in this paper,pixel-level image fusion based on Principle Component Analysis(PCA),and feature-level image fusion based on Dempster-Shafer evidence theory.In pixel-level case,the SAR image at HV polarization is relatively sensitive to the vegetation canopy.We combined the HV polarization information from SAR and spectral characteristic from SPOT images in an effort to enhance land cover classification.Before the fusion process,wavelet transform was first applied to denoise the SAR image which suffers from speckle contamination due to coherent process.PCA method is then used to fuse the SPOT and SAR images.In doing so,the PC-1 component is replaced by SAR image(approximation image,after wavelet transform) and then the inverse transform is followed.At last,the maximum likelihood classifier was used for both SPOT-XS images and fusion images.In feature-level case,fully polarization information from SAR is used to combine with spectral characteristic from SPOT images,mainly to enhance land cover classification.We first denoise the SAR image by Lee filter.Next,the maximum likelihood classifier based on different distribution was used for SAR and SPOT images(Based on Wishart distribution and multivariate Gaussian distribution respectively),to extract the conditional probability of each pixel for each class.Dempster-Shafer evidence theory is then applied,to combine the classified results of SAR and SPOT data.Experimental results show that the classification accuracy is dramatically improved by making use of the proposed methods.Data fusion can take advantage of the use of complementary information to obtain a better overall accuracy than using single data source only.