下载中心
优秀审稿专家
优秀论文
相关链接
摘要
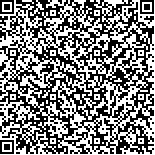
利用遥感影像对地物进行分类识别时,既需要考虑地物波谱信息,也需要考虑其空间信息。现有遥感分类方法主要集中在利用像素的波谱信息,对各个像素进行独立分类,忽略了地物空间信息。考虑到传统最大似然分类(MLC)方法包括先验概率和条件概率密度函数两个核心环节,提出基于空间信息的浮动先验概率MLC方法,融合空间信息和波谱信息,以提高分类精度。在分析地物空间信息的基础上,总结了基于空间信息的浮动先验概率确定原则和依据,包括地物几何空间特征、情景特征、临近像素空间自相关定律、景观参数等,并设计了基于地物空间特征和临近像素空间自相关定律的浮动先验概率确定算法和分类流程。通过分类试验和误差矩阵分析,结果表明:基于空间特征和临近像素空间自相关定律的浮动先验概率MLC方法,能够融合地物的波谱信息和空间信息,克服最小距离、MLC等基于像素波谱信息的分类方法的缺点,显著提高地物分类精度。
The spatial and spectral information in remote sensing data should be exploited to improve classification precision.Unfortunately,the spatial information is neglected in most traditional remote sensing classification methods.Considering two stages probability method of maximum likelihood classification(MLC),this article proposed a new method of exploiting spatial information to improve classification rules by adjusting the prior probability according to the local spatial information.The local or global spatial features of typical ground targets were analyzed,and there were four principles proposed to decide the prior probability,including spatial geometrical features,local spatial autocorrelation law,contextual knowledge,and landscape parameters.An algorithm was designed to exploit the spatial features and prior knowledge to adjust the prior probability of MLC.The experimental classification was carried out,the classification error matrices and its precision results showed that the floating prior probability MLC method proposed in this article could integrate ground targets' spatial knowledge with spectral information,and overcome the flaws of traditional pixel-based classification methods,such as minimum distance,MLC,and that the classification precision was improved greatly.