下载中心
优秀审稿专家
优秀论文
相关链接
摘要
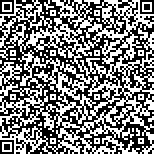
近几年来,离散小波变换在遥感图像压缩、消噪和融合中得到了广泛的应用。利用航空飞行的高光谱图像数据,先后进行了主成分分析及10种小波变换,并应用分类回归树对其进行分类处理。将小波变换的分类结果与主成分分析的结果及不同的小波变换方法之间进行了对比。结果表明,在样本数相同的条件下,小波变换的分类精度均高于主成分分析,其中Haar小波的分类精度最高;小波变换后的分类对样本数量的要求要小于主成分分析。在样本数足够的情况下,主成分分析数据压缩率要高于小波变换。但小波变换在压缩的情况下,仍保留了原光谱的大部分信息。而主成分分析只保留了原图像的方差而无法保留波形。
Discrete Wavelet Transform(DWT) was widely used in the application of Remote sensing image compression,noise reduction and image fusion recently.Principal Component Analysis(PCA) and DWT are performed separately with airborne hyperspectral data before a classification and regression tree are applied.The classification results of DWT are compared with PCA and with different mother wavelet DWT themselves.The results indicate that,when the number of the training samples is equal,the classification accuracy of DWT is higher than PCA,among them Haar wavelet performs best.The demanding number of samples of classification after DWT is less than PCA,but when the samples are enough,the compression ratio of PCA is higher than DWT.However,when DWT compression is performed,the most of the spectral information is preserved while PCA keeping the variance of the image only.