下载中心
优秀审稿专家
优秀论文
相关链接
摘要
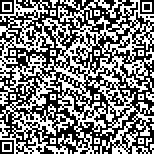
在二维马尔可夫随机场模型的基础上,提出顾及波段间的空间相关性,发展了一种适用于多光谱纹理影像分类的广义马尔可夫随机场模型。鉴于广义马尔可夫随机场模型的复杂性,利用最大伪似然法建立了求解模型参数的简化方程式,实现了纹理特征的快速提取。结合提取的纹理特征影像和光谱特征影像,采用概率松弛算法实现影像的分类。实验证明,提出的基于广义马尔可夫随机场的多光谱纹理影像分类算法克服了传统的基于光谱特征的分类算法的局限性,提高了纹理影像的分类精度。
Texture plays an important role in the composition of natural images and its analysis and classification are essential in a variety of image processing application.The method of texture analysis chosen for feature extraction is clearly critical to the success of the texture classification.Markov random fields(MRF) are a popular statistical model for textures.They capture local characteristics of an image by assuming a local conditional probability distribution.Many models used for gray level images have been proposed,but they cannot perform well in multispectral textured images.In this paper,a universal MRF model for multispectral textured images is developed,which take into account not only the spatial interaction within each of the multispectral bands,but also the interaction between different bands.As we all know,A MRF-based approach employs MRF model parameters as texture features to discriminate different textures.Because of the interaction between different bands,the universal MRF model is very complex,and estimating the corresponding parameters is very difficult.Therefore,in order to compute the universal MRF is parameters efficiently,a simplified equation using the maximum pseudo-likelihood method is built.After texture feature extraction,a supervised classification is applied to the original spectral bands combined with textural images.In this supervised classification system,the feature values are used by a Bayes classifier to make an initial probabilistic labeling.The spatial constraints are then enforced through the use of the Peleg's Probabilistic relaxation algorithm.Necessary experiments are performed on samples of FG Forrest and QuickBird imagery,and the results indicate that the proposed algorithm provides better classification accuracy than other conventional approaches.