下载中心
优秀审稿专家
优秀论文
相关链接
摘要
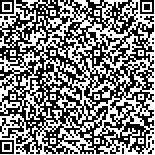
提出了一种基于人工免疫系统(ArtificialImmuneSystem)的分类方法。该方法首先应用人工免疫系统的克隆选择算法对样本进行自学习,得到全局最优的聚类中心,然后利用学习得到的聚类中心对整幅影像进行分类。由于人工免疫系统继承了生物免疫系统的自组织、自学习、自识别、自记忆的能力,从而使得人工免疫系统具有非线性的分类能力,并能够快速准确地得到全局最优解,克服了传统分类方法约束条件多,容易陷入局部最优的缺点。实验结果证明,该算法分类精度上优于传统的分类方法,总精度和Kappa系数分别达到了89.80%和0.8725,因而具有实用价值。
In this paper, some initial investigations are conducted to employ Artificial immune system(AIS) for classification of remotely sensed images. As a novel branch of computational intelligence, AIS has strong capabilities of pattern recognition, learning and associative memory, hence it is natural to view AIS as a powerful information processing and problem-solving paradigm in both the scientific and engineering fields. Artificial immune system posses nonlinear classification properties along with the biological properties such as self/nonself identification, positive and negative selection, clonal selection. Therefore, AIS, like genetic algorithms and neural nets, is a tool for adaptive pattern recognition. However, few papers have concerned applications of AIS in feature extraction/classification of aerial or high resolution satellite image and how to apply it to remote sensing imagery classification is very difficult because of its characteristics of huge volume data. Remote sensing imagery classification task by Artificial immune system is attempted and the preliminary results are provided. The experiment is consisted of two steps: Firstly, the classification task employs the property of clonal selection of immune system. The clonal selection proposes a description of the way the immune systems copes with the pathogens to mount an adaptive immune response. Secondly, classification results are evaluated by three known algorithms: Parallelepiped, Minimum Distance and Maximum Likelihood. It is demonstrated that our method is superior to the three traditional algorithms, and its overall accuracy and Kappa coefficient reach 89.80% and 0.8725 respectively.