下载中心
优秀审稿专家
优秀论文
相关链接
摘要
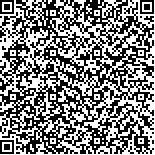
预测树方法是一种有效的无损多光谱图像压缩技术,将自适应线性预测方法与传统预测树方法相结合,提出了一种多光谱遥感图像的误差补偿预测树压缩方法。该方法利用多光谱图像谱间的局部统计冗余和结构冗余建立自适应预测器,对传统预测树方法产生的误差进行补偿,从而进一步减少了多光谱图像的数据量;并且利用多光谱图像的局部平稳特性对算法进行了简化。实验结果表明,该方法得到的压缩比与原始预测树方法相比有明显提高,同时算法简化后可以使计算复杂度大幅度降低。
A novel error compensated prediction tree method used for lossless compression of multispectral imagery is proposed in this paper. This method combines prediction tree and adaptive linear prediction techniques, so the spatial redundancy, spectral statistical redundancy and spectral structural redundancy in multispectral imagery are all exploited. For each pixel of the imagery, the prediction tree based on interband structural similarity is used to remove the spatial redundancy and a prediction error is created. Then this error is compensated by an interband linear adaptive predictor, which is constructed according to the spectral statistical redundancy. Our method removes the spectral structural and statistic redundancies as well as the spatial redundancy, so the better compression results can be obtained. The construction of adaptive predictor for each pixel will introduce prohibitive computational complexity to the algorithm. Therefore a modified algorithm based on local stability of imagery is also designed to reduce the computational complexity. The experimental results from practical multispectral images have shown that our method is better than the original prediction tree one.