下载中心
优秀审稿专家
优秀论文
相关链接
摘要
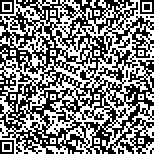
提出了一种基于数学形态学算子的多尺度聚类方法 :首先将数据进行二值图像化处理 ,利用一次闭开运算去除噪声干扰后再利用逐步增大结构元素的闭运算构建尺度空间 ,图像内的连通单元集随着尺度上升不断融合 ,最终全部归并。将连通集覆盖下的点集归为一类 ,以上步骤就对应了一个多尺度层次聚类过程。本算法的一个最大优点是聚类个数事先无需设定 ,而被确定为跨越最多尺度 (具有最长尺度生存期 )的类别个数。此外 ,参数少、能够提取任意形状的类别、具有较强的抗噪声能力也是算法的优点。对自构建数据与地震数据的聚类实验验证了方法的有效性和实用性。
关键词:
数学形态学,尺度空间,聚类In this paper, a scale space clustering algorithm based on mathematical morphology operators(MSCMO) is proposed. The data are firstly converted into a binary image, the noises are then deleted with close open operators. A scale space is constructed with the close operator and structure elements as well as increased size. The connected cells merge with each other with the increasing scale until all of them combine into one. We suggest this is just a multi scale hierarchy clustering process considering the data under the connected cells into one class. One of the biggest advantages is that we do not need to set the cluster number before hand, it is fixed in the end on the cluster number which spans the longest scale range (with the longest`scale survival time'). Besides, less arguments the ability to extract clusters with arbitrary shapes, and the robustness against noises are also the advantages of MSCMO. The validity and practicality of the algorithm are validated with constructed data and earthquake data.