下载中心
优秀审稿专家
优秀论文
相关链接
摘要
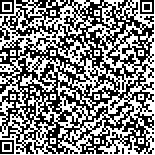
遥感反演大多是典型的约束是最优化问题。本文对现有的约束最优化方法在遥感反演中的适用性进行了分析,从提高反演速度有降低优化方法病态特性两个方面考虑,提出了罚矩阵的概念,对约束优化方法中的乘子法进行了拓展,并进行了理论证明。经过大量的模拟反演实验表明,拓展后的乘子法的反演速度提高了大约30%,病态程度也得到了进一步的改善。
Inversion algorithms are very important in quantitative remote sensing. Currently, the classic least square method is still used widely. We suggest that remote sensing inversions are often typical constrained optimization problems. Many good constrained optimization methods may be used in remote sensing. After a brief review of the constrained optimization methods, we discuss the widely used augmented Lagrange multiplier method in detail. Only one penalty factor is used in this method, even if this factor is not required to be infinitive in theory, it may still increase larger and larger to meet several constraints with very different magnitudes. As a result, similar to the penalty function method, the ill-posed problem and low efficiency still bother the augmented Lagrange multiplier method. As a solution, we extend the penalty factor to be a diagonal penalty matrix, and present an extended augmented Lagrange multiplier method. Because different constraints are given different penalty factors in this new method, a priori knowledge can be used to help decrease the ill-posed problem and increase the iteration speed. After proving this new method in theory, we do detailed simulation and inversion as further validation. It is clear from the statistical analysis that the rate-of-convergence of our method has been improved of about 30 percent compared with the original penalty factor based method but with similar accuracies. Furthermore, it is also found that our extended method is resistant to ill-posed problems.