下载中心
优秀审稿专家
优秀论文
相关链接
摘要
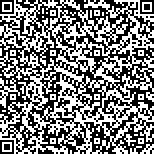
介绍了一种基于人工神经网络的海中一类水域叶绿素反演方法。人工神经网络是3层的反向传输神经网络。其结构是输入层有4个节点,它们分别对应4个波段412m,443nm,490nm,510nm的遥感反射比与555nm波段遥感反射比的比值,隐含层有5个节点,输出层一个节点对应于叶绿素深度。该神经网络的训练和试验样本集来自SeaBAM的数据集。数据集中的919个站位的70%(644个)用于训练,30%(275个)用于测试。结果表明,该方法的精度优于被广泛采用的三次经验方法。
A method to retrieve the oceanic chlorophyll concentrations in Casse I water based on Aritificial Neural Network (ANN) is presented. The ANN used in this paper is a three_layer feed forward back_propagation network which has 4 neurons in the input layer (corresponding to the ratios of the remote sensing reflectances at 4 wavelengths: 412 nm, 443 nm, 490 nm, 510 nm to the remote sensing reflectance at 555 nm), 5 neurons in the hidden layer and one neuron for the output layer (Corresponding to chlorophyll concentration). The training data set and testing data set of the ANN come from SeaBAM data base. 70% of the 919 stations in SeaBAM was used for training data set, the other 30% used for testing data set. At the end, the retrieved results from ANN and from the empirical algorithms were compared. The results show that the accuracy of the ANN is better than the cubic empirical algorithms used widely.