下载中心
优秀审稿专家
优秀论文
相关链接
摘要
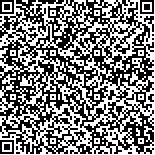
本文根据植被类型分布与地理环境因子的关系, 在地理信息系统和遥感技术支持下, 通过GIS叠加、统计分析操作, 建立植被分布与年积温、降水量、海拔高度、土壤类型等环境因子的定量化知识向量表。综合应用所得到的地学知识向量表和植被光谱特征值进行分类试验, 得到研究区的植被分布图。文章以贺兰山地区为例, 详细介绍该方法的应用。
There are many c1assification methods for thematic mapping using satellite remote sensing image. The accuracy of c1assification is limited and unsatisfied. Here,a knowledge-based vegetation classification is presented, which inc1udes two main steps: extracting knowledge and classification based on knowledge. The first step is that the knowledge is extracted by analyzing the relationship between the relative environment factors and each kind of vegetation supported by GIS.The distribution maps of several environment factors, inc1uding soil, elevation, temperature,precipitation, are selected and are overIapped respectively with vegetation distribution map of previous period. As far as the soil factor, which can not be described with numerical value, the Bayes theory was introduced to describe the probability of the c1assification. On the other hand,for the factors inc1uding elevation,temperature and precipitation described with numerical value, a new method was introduced to describe the c1assification probability.In the end,a vector expression is designed to describe the relationship between each kind of environment factors and each kind of vegetation through statistical method. Thus, the knowledge (the vector expression) is extracted. The second step is that a method based on knowledge derived from above is designed to classify the vegetation for remote sensing image.The difference between this method and other methods is that the knowledge is brought into play as assumed several bands of spectrum.During the c1assification procedure,the contextual check was made to ascertain whether the pixels had been c1assified into the vegetation types or not that were ecologically valid for the grid cell being considered. After used the knowledge, the accuracy is increased obviously. To surnmarize, the classification accuracy was increased about 9 percent using the knowledge-based method presented,compared to the supervised classification method that does not use knowledge.