下载中心
优秀审稿专家
优秀论文
相关链接
摘要
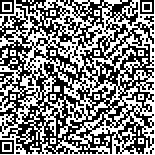
利用高分辨率光谱仪在实地测得的光谱数据来识别美国加州的6种主要针叶树种。树冠阴面和阳面的高光谱数据分别在1996年夏、秋测得。首先对原始光谱数据作简单处理, 然后进行6种数据变换:对数变换、一阶微分变换、对数变换后一阶微分变换、归一化变换、归一化变换后一阶微分变换及归一化后对数变换。采用相邻窄波段逐步加宽的办法, 测试不同波段宽度对树种识别精度的影响。所有的变换方法及波段宽度试验最后均由神经元网络算法产生的树种分类精度来评价。试验结果表明对数变换后一阶微分和归一化变换后一阶微分能够获得高于94%的平均精度
In situ hyperspectral data obtained with a high spectral resolution radiometer were analyzed for idendfication of six conifer species. Hyperspectral data were measured in the summer and late fall seasons from both the sunlit and . shaded sides of canopies. An artificial neural network algorithm was applied for the identification purpose. Six types of transformation were applied to the hyperspectral data R preprocessed with a simple smoothing followed by band merging. These include log(R), first derivative of R, first derivative of log(R), normalized R, first derivative of normalized R, and log(N(R)). First derivative of log(R) and fist derivative of normalized R resulted in best species recognition accuracies with greater than 94% average accuracies. The effect of hyperspectral data taken from the shade sides of tree canopies can be minimized by applying normalization or by taking derivative after applying logarithm to the preprocessed data. We found that a big difference in solar angle due to seasonality did not cause noticable difference in accumcies of species recognition. A band width of 20nm or narrower is recommended for the recognition of the six species.