下载中心
优秀审稿专家
优秀论文
相关链接
摘要
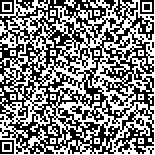
该文提出一种由多层神经网络与自组织神经网络相结合进行多类别遥感图象分类的复合神经网络分类方法。第1步将训练样本按其统计特征分成若干组,用不同组别的训练样本分别训练BP网络。第2步将这些训练好的BP网络并联构成有监督分类器,对遥感图象进行有监督分类。第3步用BP网络的分类结果对Kohonen网络进行自组织训练,用训练好的Kohonen网络构造无监督分类器,对遥感图象进行细分。通过对SPOT遥感图象的分类实验表明,该方法对多类别遥感图象很适用,能显著提高分类的数目和精度,对一幅SPOT遥感图象进行的分类实验,结果可分类别数高达48类,对其16大类的有监督分类的精度可达91.6%。
This article presents a new approach to pattern recognition of multicategory remote sensing images by using multilayered neural network and self-organizing neural network. BP algorithm is a typical supervised classification method by which multilayered neural network can learn previous knowledge about patterns of remote sense image from trainning sample set and form complicated nonlinear decision function automatically. Kohonen neural network, which may produce what is called self-organizing feature maps similar to those that occur in the human brain, can be used as an unsupervised classifier. In this article, considering the features of multicategory remote sensing images, we synthesize the advantages of these two methods to form a complex classifier. First, trainning samples are divided into several groups to train the corresponding BP networks which parallel to construct supervised classifier. Second, the remote sensing image is classified into many gross classes by the supervised classifier. Third, use the result of the supervised classifier to train Kohonen network and each gross class is classified into some sub-classes by the trained Kohonen network. This method has been used in the classification of a SPOT remote sensing image, the number of recognizable classes is 48 while the average right rate of the supervised classification is 91.6%. The experimental results verify the usefulness of this approach.